Case Study: Navigating through Data Quality Challenges in Market Research
Exploring the Roadblocks to Reliable Insights and Actionable Data
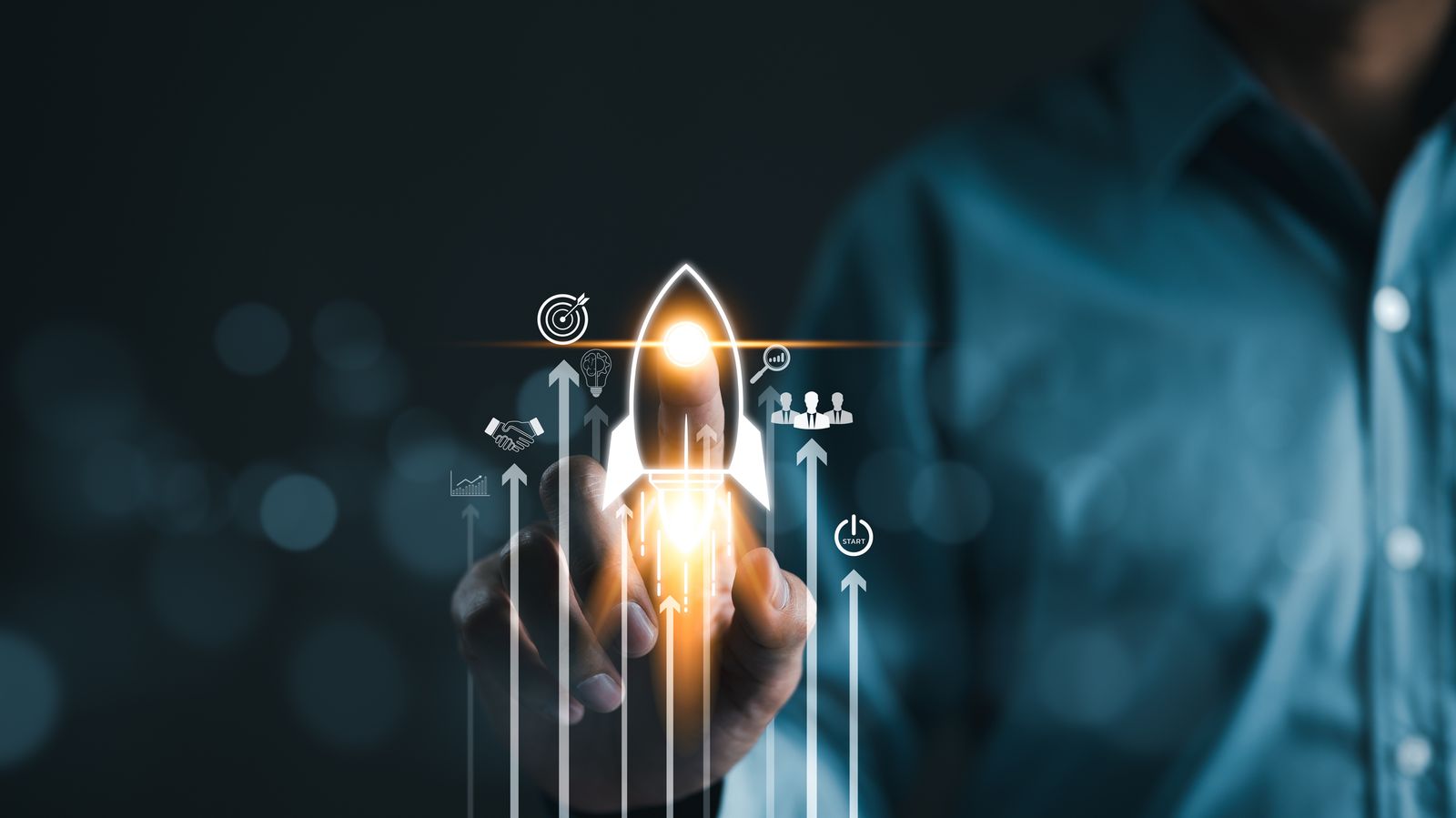
The research was conducted in March-April 2024 through the online to learn about the pain points Market Research Professionals face in ensuring data quality. The findings of this research shed light on the significant challenges faced by Market Research Professionals regarding data quality. A vast majority of market research professionals encounter various issues, with incorrect sampling methods and sampling/non-sampling errors being the most prevalent. Sample bias, data fraud, and response bias are also notable challenges, affecting around one-third to half of the professionals. Furthermore, data authenticity verification methods are found to be lacking, with only a small percentage employing essential quality checks.
This highlights a critical gap in the industry and underscores the urgent need for improved data quality standards and methodologies. Moving forward, it is imperative for Market Research Professionals to address these challenges effectively to ensure accurate and reliable data collection, thereby enhancing the quality and reliability of insights derived from market research.
Objective
The aim of study was to identify the challenges encountered by the market research industry regarding data quality. Emphasis was placed on examining issues with data collection, its authenticity, tools and techniques used to refine the data collection process and validating the data quality.
Key Findings:
· Nearly 60% of the Market Research Professionals admitted that they are facing data quality challenges majorly and 58% of the Market research top management considered the same as an issue and, one third of the market research consultants also face the data quality challenges in market research Industry.
71% Market research consultant mentioned that respondents are not reading the questions during the survey hence, the mono response rate is higher end.
Nearly half of the professionals accepts that Response bias occurs majorly during data collection due to wrong sampling method.
More than Half of the Professionals stated that Invalid responses consisting of Outlier/Junk or Mono Answers in compromising the reliability the data.
As per 63% of the Mid-Level Management surveys are not completed in the appropriate time frame due to low response rate.
3 out of 5 Market Research Professionals trust the Panel Provider Vendor Data for Data Collection purposes.
83% of the Professionals are not using any Data Quality Score Measurement model to Identify the quality of their data.
Only half of the Data Collection Agencies are using Sample Management Tools to detect Fraud detection (Multiple IPs, ISPs, Devices, Ghost Completes).
8 out of 10 Market Research Agencies are not using sample Matrix to Identify whether their panel provider is capable of providing the required sample or not, in order to improve overall sampling method and reducing sampling Bias.
Only a handful of Online Data Collection Agencies validate their data using different statistical models & Technologies to improve the quality.
The above Key Findings have helped us identify the huge gap Data Quality in the Market Research Industry.
The survey covered 18 countries and involved 103 Market Research companies and consultants, with a total of 117 participants.
Out of these participants, 37% were top and senior-level management, 32% were Market Research Consultants, and 31% were middle-level management.
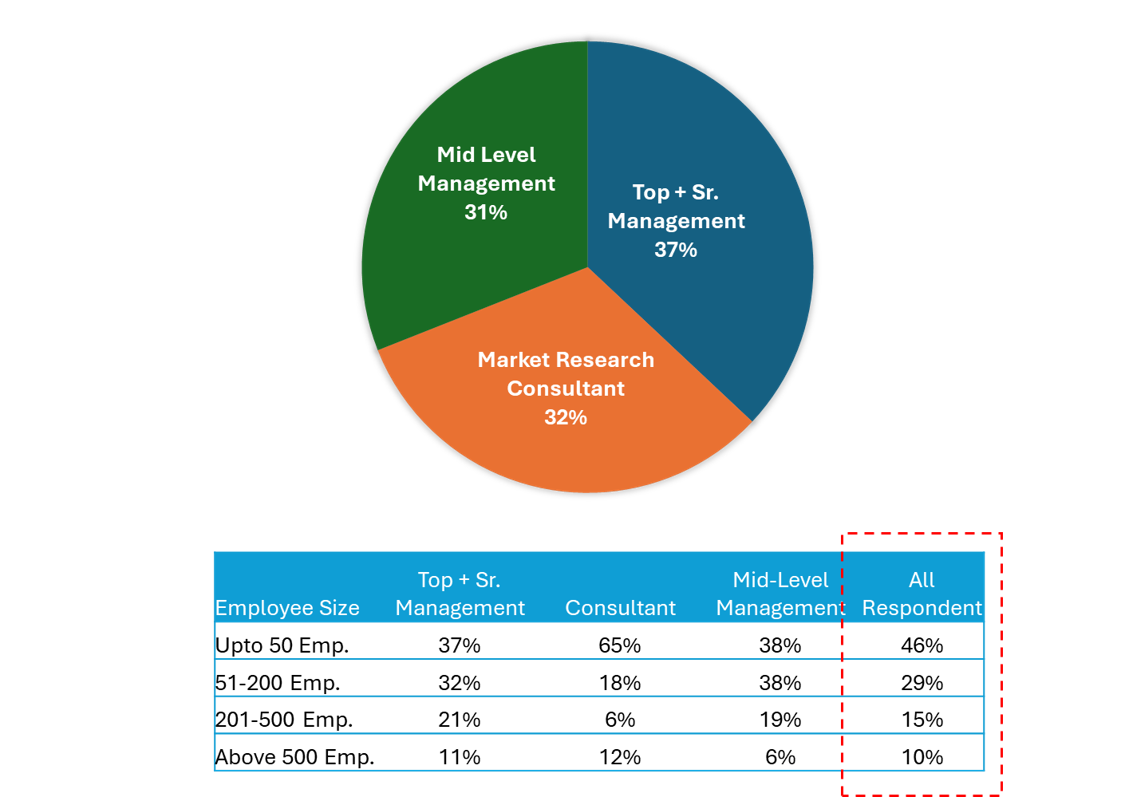
Among the participants, a quarter are working in companies with over 200 employees, while half belonged to organizations with fewer than 50 employees & lastly, one in every four participants worked in companies with 51 to 200 employees.
Various types of market research service providers were included in the study. Among them, 23% offered all types of market research services, 31% specialized in data collection, 27% provided market research consulting services, and 19% focused solely on market research analytics.
Research reveals that 60% of Market Research Professionals encounter challenges related to Data Quality. Among these, half face issues stemming from incorrect sampling methods and sampling/non-sampling errors. Additionally, one out of three professionals experience sample bias challenges during online data collection. Data fraud challenges affect 50% with more than half of the respondents being identified as outliers or providing junk and speeding survey responses.
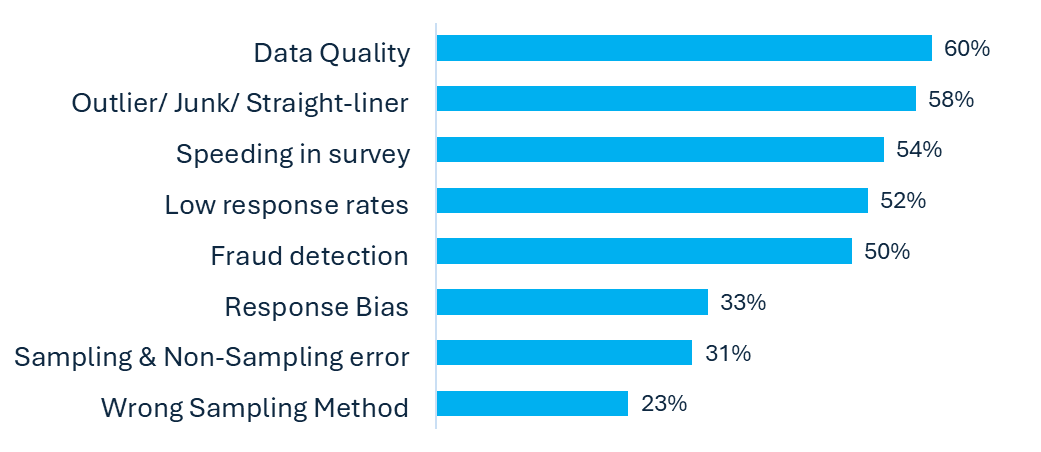
Process of Verifying the Data Authenticity
Market research professionals employ various methods to verify data authenticity. One of the common approaches is to trust a third party, and around 14% of the professionals fall into that category. Another significant aspect is to verify the quality of open-ended responses, which is performed by 15%, and only one-fifth of the professionals closely examine outliers, to ensure data accuracy.
Moreover, quality checks such as trap questions, logic checks, and attention checks play a crucial role, contributed by one-fourth of the professionals followed by comparing new data with existing datasets is another essential step, making up another 21%.
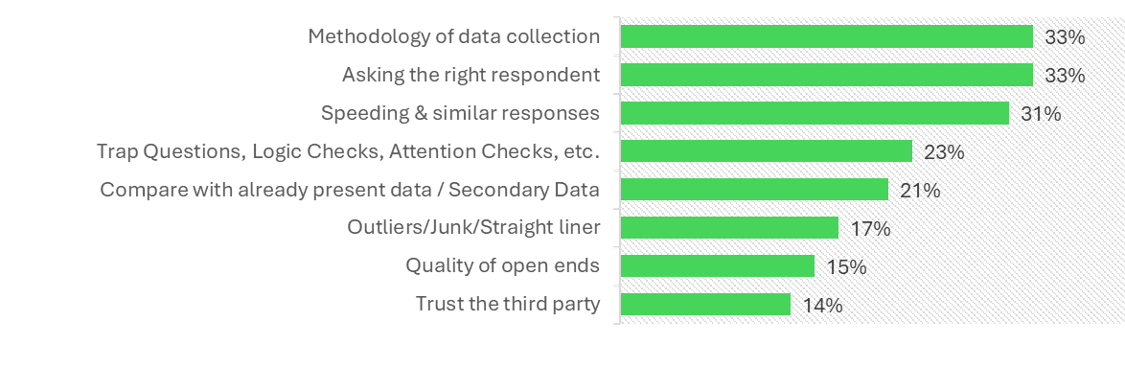
Targeting the right respondents is crucial for 33%. Meanwhile, only one in three professionals focus on identifying and rectifying speeding and similar responses. Lastly, 33% prioritize the basics of data collection methodologies to ensure data accuracy and consistency.
Almost half of the agencies overlook important aspects of data collection, such as using the right methods and choosing the right participants. Surprisingly, 3 out of 5 agencies don't perform essential quality checks like trap questions or logic checks. Also, about 50% of the agencies don't verify outliers or speeding respondents, which leads to lower data quality scores. Moreover, only 7% of data collections follow the right approach for data verification authentication, showing a big gap in the industry for improving data quality and getting better insights.
To address these challenges, the implementation of a comprehensive assessment tool is recommended. This tool should measure data quality across different dimensions, such as sampling methods, identification of speeders, mono answers, outliers, and junk in open-ended responses.
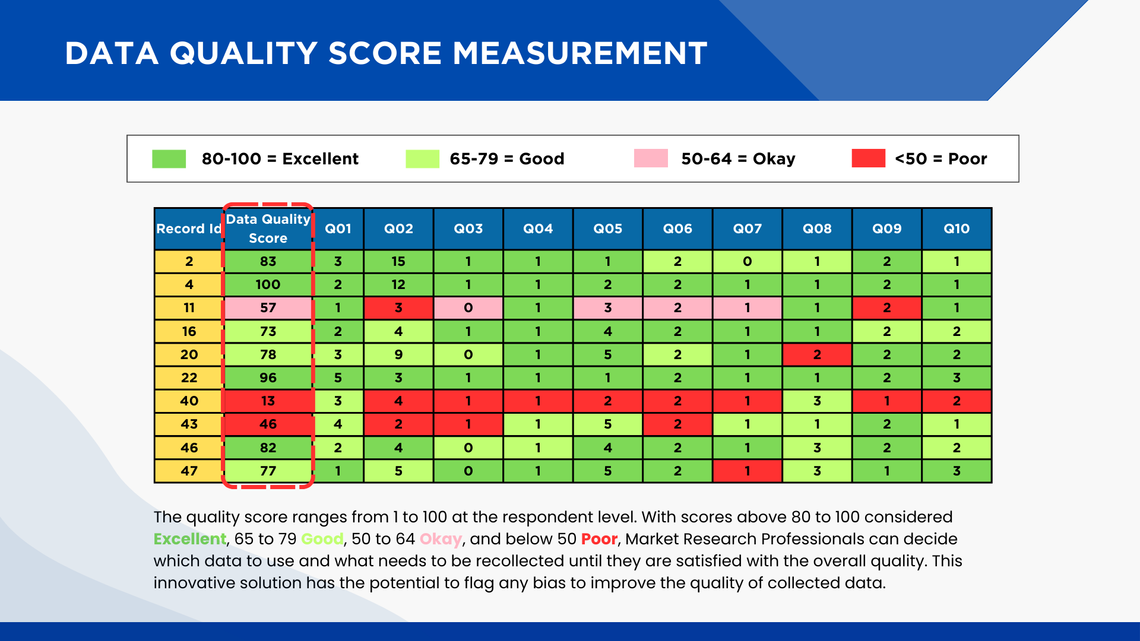
By employing a variety of methods to address common challenges, including measuring data quality across different dimensions such as the appropriate sampling method, identification of speeders, mono answers, outliers, and junk in open-ended responses, ensuring data consistency, representativeness, accuracy, and fraud detection.
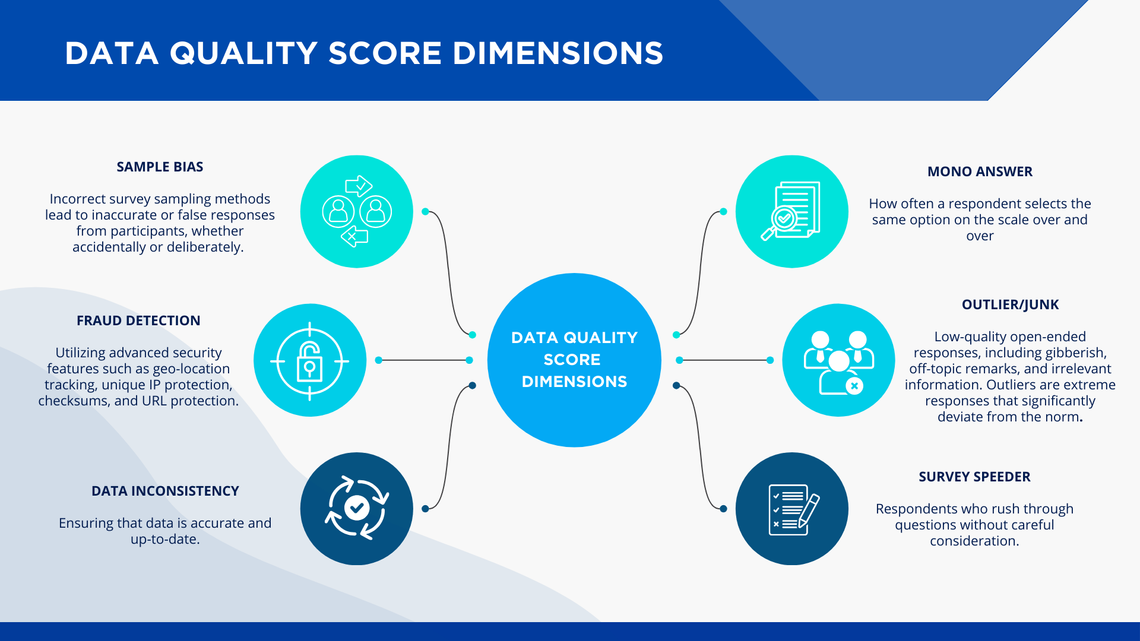
Pradyumna Parida is a dedicated and experienced analytics professional with a strong background in market research, statistical modelling, and data analytics. They excel at transforming complex datasets into actionable insights that support strategic decision-making and are proficient in a range of statistical tools and programs. Pradyumna is passionate about applying innovative research methodologies to drive business growth and deliver impactful results.
With expertise in client operations, report writing, and data visualization, Pradyumna is a skilled storyteller who combines analytical precision with creative presentation. They craft compelling narratives and impactful pitch decks, empowering organizations to make informed and confident decisions.