Beyond the hype
Innovation predictions in the era of Machine Learning
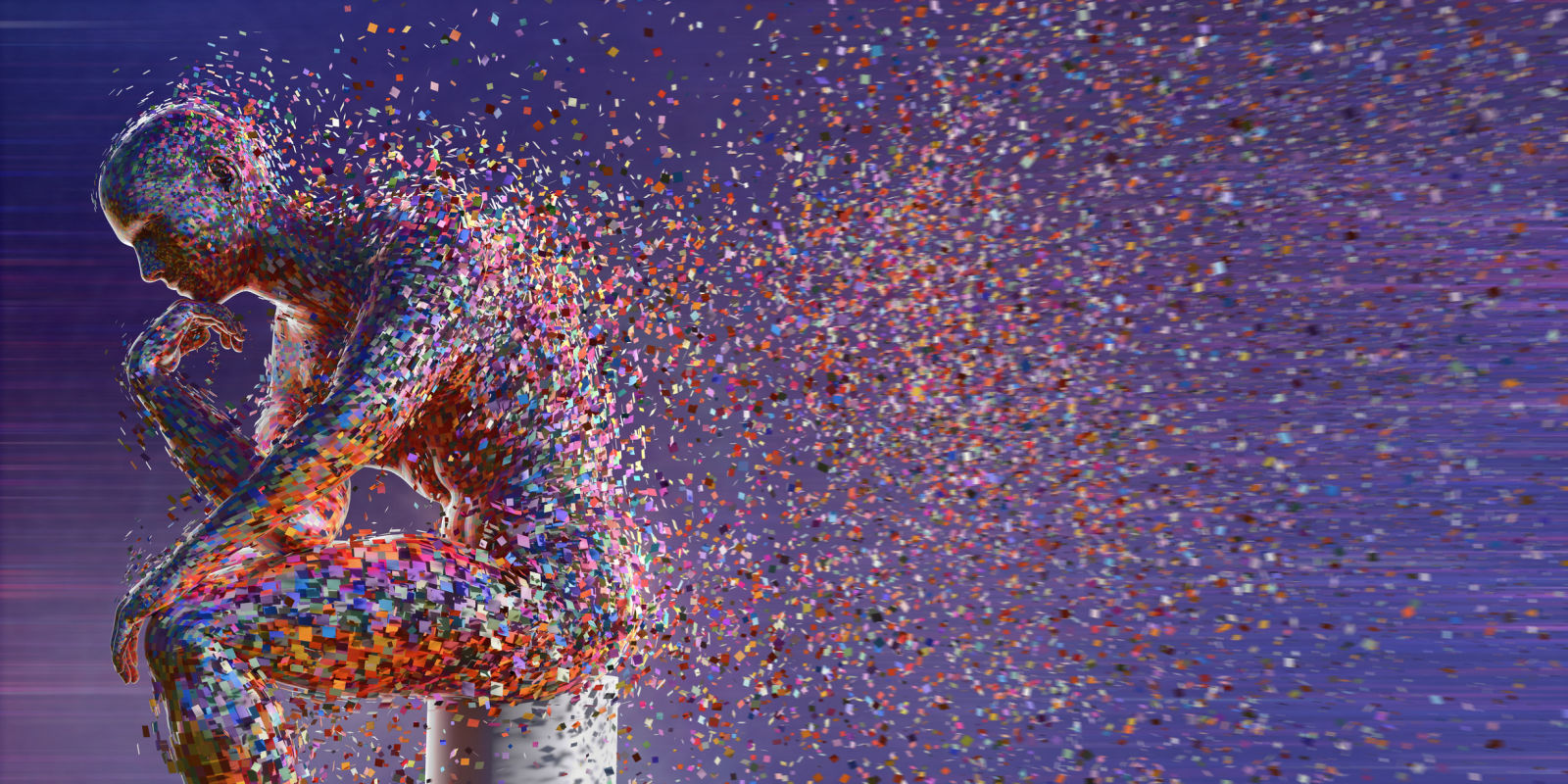
Artificial intelligence (AI) has grown in popularity in recent years. Voice and facial recognition software, for example, have been developed in many technical gadgets, and e-commerce sites have implemented AI algorithms that recommend products for purchase. In this context, we are beginning to see how AI can also alter market research, resulting in faster, cheaper, and better results. Ipsos’ Beyond the Hype article focuses on a single domain within AI: the application of Machine Learning (ML) to predict the success of new product innovations. Ipsos goes beyond the hype by helping readers understand how AI/ML models for predicting innovation success can be built and importantly, their limitations.
In a nutshell, machines need to be trained before they are useful. Without training, machines are directionless algorithms. Conceptually, training is done by showing a machine lots of examples of what you want it to predict. This is akin to teaching a young child to identify objects. For example, if you wanted to teach a child to recognize mobile phones, you would show the child examples of mobile phones and non-mobile phone objects. In doing so, the child learns to recognize the features that define a mobile phone and becomes able to identify them in the future. Training an AI/ML model to recognise an innovative product is similar. We need many examples of both successful and unsuccessful innovations so that an AI/ML model learns to recognise the traits that foretell successful breakthroughs.
Limitations
Once we realize that AI/ML models need examples to be trained on, we can understand their limitations. The predictive power of an AI/ML model is completely reliant on the examples used to train the model. Extending the child learning example, if a child is shown only standard mobile phones during the training phase (i.e., rectangular, glass screen), the child will be unable to identify new phones in a different format (e.g., as a wrist band).
The training examples used will also determine the scope of the model. Training a child to recognize mobile phones means just that – a learned ability to recognize mobile phones. If we want the same child to recognize a calculator, for example, the child will need to be provided examples of calculators. A model is only as good as what you train it on. Machines are narrow learners.
Because of the dependency on training examples, innovation prediction models trained using the content/description of previously tested concepts are not optimal for predicting the success of future innovations. Such concept-based models have two important limitations: a decreased ability to predict the success of new products that don’t exist today and narrow scope.
Think about how quickly products evolve over time (e.g., the evolution of land-based phones to mobile phones) or how quickly consumers’ needs can change due to market disruptions (e.g., climate change, Covid-19, price inflation, supply chain issues). Concept-based predictive models may perform well for a year or two but will quickly become obsolete due to market changes or changes in consumers’ needs. When concept-based models perform well, it is also likely only for items that are like those already on the market rather than truly innovative ones. AI/ML models tend to predict best when the new stimuli are like the examples used for training. This limits the ability of concept-based models to predict the success of new products that don’t exist today.
Concept-based models are also likely to be limited in the scope of applicability. If an AI/ML model is trained using previous food concepts, for example, we would not be able to use the same model to predict shampoo concepts (since these concepts would have very different product descriptions). Similarly, if an AI/ML model has been trained using US food concepts, we would not expect the model to work for non-US countries (e.g., China) as food preferences can vary considerably across countries.
Using consumers for training
At Ipsos, we use consumers’ reactions to new products to train AI/ML models to predict the success of new products. By consumer reactions, we mean the immediate responses consumers have after seeing a new product concept. This immediate response is critical as it determines whether consumers want to learn more about a product and eventually purchase or disengage. We use consumers’ reactions to train our AI/ML models because we believe that consumers’ responses are robust and stable predictors of innovation success over time and because AI/ML models built on such data may have broader applicability across markets and categories.
Consumer's responses to new product ideas are stable because how consumers respond to new products today will likely remain similar in the future (e.g., positively, negatively, with scepticism). These responses to new product ideas are often also universal. That is, regardless of the country consumers live in or the specific product they are reacting to, there are reactions common across all markets and product categories: positivity, negativity, scepticism, indifference, confusion, etc. The universality of such responses allows for more scalable models that can be applied across markets and categories.
Colin Ho
Chief Research Officer at Ipsos, Innovation and Market Strategy and Understanding at IpsosConsumer insight executive with a unique amalgamation of research, data science, psychology, and management consulting skills. Proven successful at extracting and communicating deep consumer insights for clients, and at developing global research innovations by interweaving research analytics, consumer psychology and strategic consulting together