Big data analytics: Role of automation
Thanks to automation, data analytics has also become more accessible and cost-effective thanks to automation.
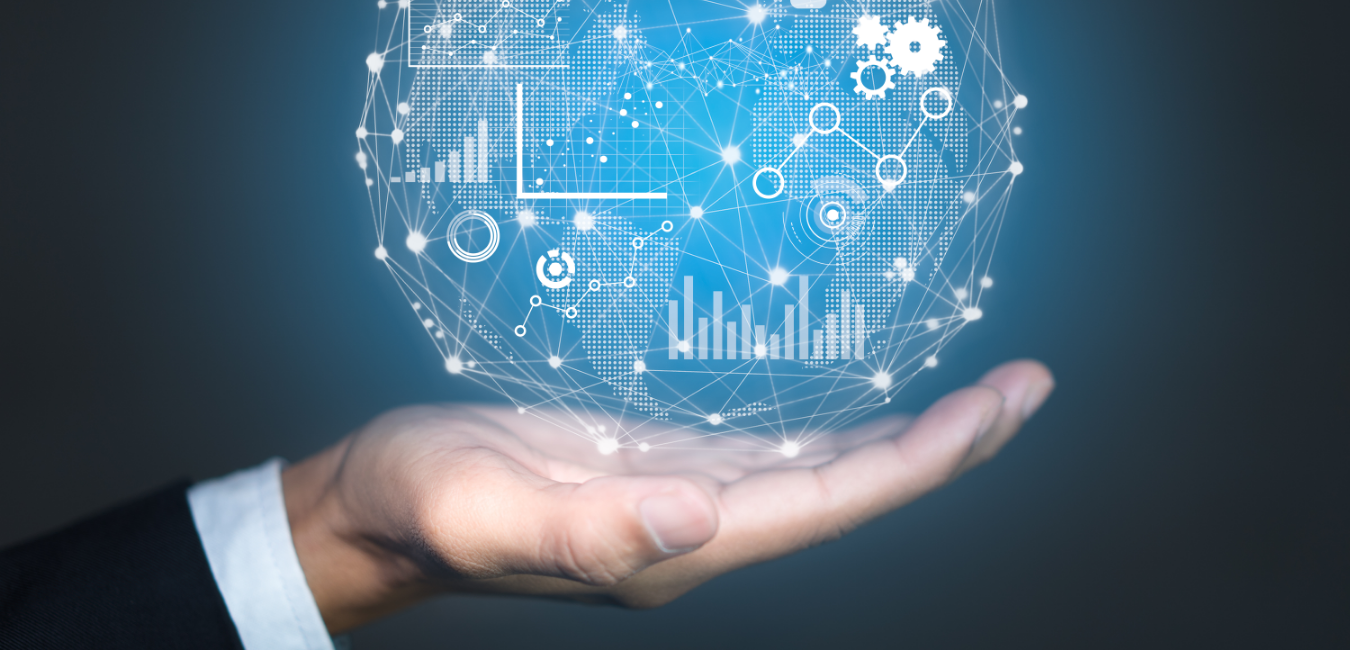
The process of examining datasets to conclude the information gathered is called data analytics. It involves a combination of various techniques and processing methods. The techniques use specialized systems and software that integrate automation.
These techniques are used by data scientists in their research activities. Businesses use techniques in data analytics to make informed decisions. It enables them to understand their clients, assess ads, and develop products. Simply put, businesses use data analytics to improve performance.
Data analytics also enables organizations to use their data in identifying new opportunities. This, in turn, contributes to smarter business moves, efficient operations, and improved profits.
Before data analytics, business intelligence solutions were used to extract and load data. The challenge was that the database technology was unable to handle many streams of data at a time. As a result, it couldn’t change the input information in real-time. Neither could the reporting tools handle anything other than a relational query.
But with the entry of big data solutions, organizations can now make better decisions.
Role of software in big data analytics
Big data analytics software enables users to gain insights into large data sets collected from big data clusters. These tools enable organizations to digest data trends, anomalies, and patterns. Team members are also able to understand data visualizations, dashboards, and reports.
Big data analytics enables software development companies to understand what works and what doesn’t. During software development, big data analytics plays a big role in finding out trends and patterns. This enables developers to come up with a product that’s well-tailored to the users.
Data analytics enables software developers to analyse every feature of the software in terms of how users interact with it. Humans can manually do this but it can take a lot of time. With automated software testing, this allows organizations to use software tools that do this automatically.
To boost productivity, organizations should let team members learn automation testing.
Organizations must be able to run tests automatically, efficiently manage test data, and use the results to improve overall software quality. Automation testing makes all of this a possibility.
It’s like a quality assurance measure. But, it has to involve the commitment of the whole software development team.
Automation testing comes with many benefits. It takes care of tedious cases as well as managing broad and expansive cases. It also takes care of repetitive tasks. This, in turn, reduces business costs, saves on time, and improves accuracy.
There are many automation testing tools and the task you’re performing determines the tool you’ll use. Some of the automation testing tools are Mabl, TestProject, TestingWhiz, Selenium, Testim, and Squish. These tools allow organizations to perform tasks with minimal human oversight.
Let’s now get into a detailed discussion of the role of automation in big data analytics.
1. Democratization
Democratization of data means that data is made available, not only to analysts and the top brass but to everyone. This enables employees to understand the data they’re accessing. They’re also able to influence business decisions and expand opportunities.
Analytics and data science used to be functions exclusively meant for experts. But now users can analyse data with the help of the right analytics automation platform.
If you were in HR, marketing, or financial analysis, you have had to turn to IT. The other option was to hire experts to carry out data analytics. Today, analytics automation has increased the power of cloud computing and open source. They have also enabled access to machine learning abilities that have democratized analytics.
Thanks to the analytics automation platform, users can drag and drop automation blocks in a palette. This provides instant insights. The potential that comes with automation is huge. It relieves data workers from unproductive time spent on data discovery.
Those in favour of the democratization of data believe it is important for businesses to be transparent about their data. Because it gives a business a competitive advantage.
One of the benefits of democratization is that it empowers employees with self-service analytics. For better use of data, employees receive training in data governance.
The limitation of democratization is that organizations have to start from scratch. They need software to manage all the data. They’re also required to train employees to handle the software.
2. User experience
User experience has been getting the recognition it requires for some time now. The market now has easy-to-use phone applications and convenient features. Consumers can now enjoy better apps that come with B2B productions as opposed to what they experienced with B2C settings.
Users prefer simple and engaging interactions with their analytics tools. And this is exactly what they are getting with automation platforms. They’re giving them the code alternatives they need.
Expertise in analytics is no longer a must-have requirement. This is because of the automation tool’s ability to let you move from data to insights with ease. From creating macros to creating parameterized analytical applications.
Automation has enabled users to focus on practicing good data storytelling. This involves putting data elements in a way that creates a cohesive narrative. They can also concentrate on the most important insights of the business.
Cloud computing comes into mind when people think of big data trends. When cloud computing is merged with big data, the user experience gets enhanced. A lot of brand interaction is also happening through digital services. Thus, organizations must advance updates. They should also deliver novel products and services much faster.
3. Improving data quality
Data quality is everything. If you’re using existing data to train a model without cleaning that data, you’ll certainly get a bad model. The model may be good. But, if you put poor-quality data into it, the results won’t be pleasing.
The use of poor-quality data causes consumers to lose confidence in you. It also causes a big financial impact on a business. Poor quality data is estimated to have a financial impact of $ 15 million per year on an organization.
So, how do you define what is and what isn’t quality data? The answer to this question depends on the problem you’re trying to solve.
Data preparation is a critical aspect in identifying data quality issues. It ensures that data repair happens in good time and this is where automation comes in. Data analysts can reduce the time they spend on preparing data with automation.
There are several products built to automate data collection, cleansing, and other tasks. Robotic Process Automation is software that ends repetitive tasks. With it, organizations can extract data from different systems. It also enables initial quality checks and compiles data into a single file. Data quality is measured based on four primary dimensions:
Accuracy
Consistency
Completeness
Timeliness
RPA automates the digitization and collection of data, which guarantees high data quality. Data extraction and synchronization are popular ways of automating data management.
Apart from extracting and preparing data, automation improves underlying data quality. It does this by avoiding the errors introduced by manual data entry.
4. Data analysis from any system
Some organizations rely on legacy systems that don’t have APIs such as mainframes. The problem with this is the challenge that comes with extracting data for analysis. It also requires manual work oftentimes.
Organizations can extend the data reach of analytical tools into legacy systems without APIs. Automation enables the extraction and analysis of organizational information. It also consolidates unstructured data into a single data source, ready for analysis.
Reduction of errors is one of the benefits that come with RPA. This enhances customer satisfaction with lesser errors. RPA also enables overtime minimization of data analysis staff. This paves the way for more saved expenses. The other advantage is that thanks to RPA, users get the insights needed at a faster rate. This enhances efficiency.
5. Smarter, responsible, scalable AI
Data experts know that data and platform capabilities with automation deliver good results. For better learning algorithms, organizations need smarter, responsible, and scalable AI. So, organizations have to figure out how to scale technologies.
Because traditional AI techniques depend on historical data, they may not be relevant. The pandemic has changed how businesses operate. For that reason, AI must be able to operate with fewer data techniques and adaptive ML.
AI systems can also protect the organization’s privacy and comply with federal regulations.
6. Improved productivity
Before automation, employees used to do a lot by themselves when it comes to big data analytics. With the changing technological times, they can now relegate those tasks to computers. This makes work faster, it’s also done more accurately and saves the organization money.
Generally, automation gives employees time to take care of complex and insightful tasks. Their time gets freed up, allowing them to focus on tasks such as interpreting the automated data. They’re also able to develop new courses of action based on automated data.
It’s safe to say that automation in big data analytics leads to new products, and tweaks the existing ones. This makes them more affordable and useful. Which increases an organization’s competitive edge in the market.
Final thoughts
The integration of automation in data analysis is very important for organizations. This is considering all the benefits outlined in this article and many others.
Automation in data analysis is a huge step towards improving employees’ efficiency in today’s world.
Thanks to automation, data analytics has also become more accessible and cost-effective thanks to automation.
Daniel Martin
Co-Founder at LinkDoctorAviation technologist turned digital marketer. Love people-centric leadership.
I find immense joy in building winning teams that solve big problems while lifting each other and lead by example. My focus is on bringing innovation into the workplace culture and strategy while finding simple ways to solve complex problems.
My work has taken me across continents and allowed me to build friendships with people from all walks of life. I’ve worked for a major corporation in the UK. I've worked with people from the UK, US, Japan, France, Italy, Philippines, Hong Kong, and Singapore.
Core Beliefs: Love One Another