The unreliability and invalidity of synthetic respondents - Part Two
Part two in a three-part series that explores synthetic respondents and their impact on the market research industry.
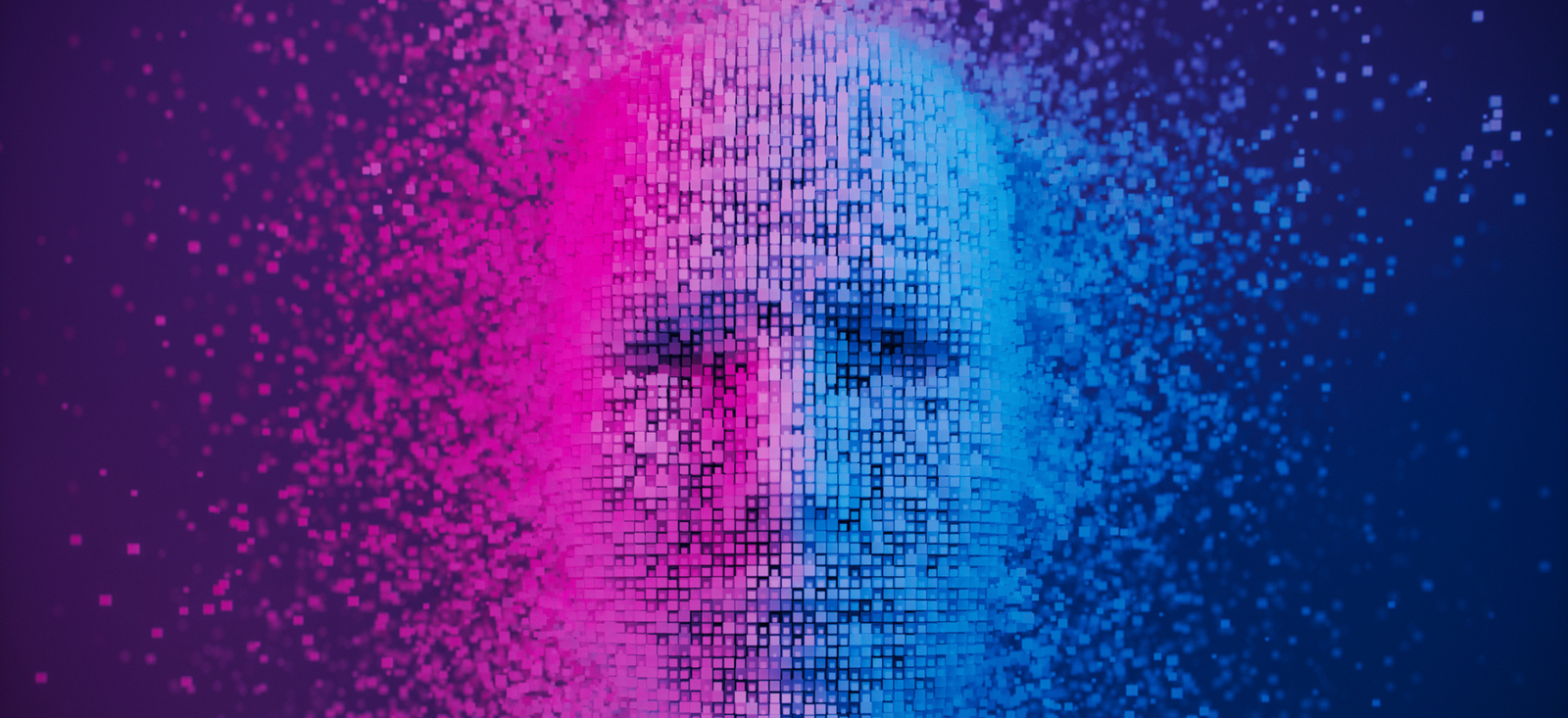
Synthetic respondents generate unreliable results and face numerous sources of error, making them unsuitable for legitimate market research purposes.
In the first article of this series, I introduced the concept of synthetic respondents and how they are generated. In this article, I will demonstrate the unreliability and invalidity of these fake responses, and discuss the different sources of error involved.
A demonstration of test-retest unreliability of fake responses
Let's do a demonstration and have GPT 4, not fine-tuned on any particular answers, complete a short questionnaire:
What is your annual household income? (Insert only USD numerical integer values)
What do you have for breakfast? (Open-ended response)
What type of toilet paper do you prefer the most? (Choose strictly one option)
Soft bleached
Standard bleached
Soft non-bleached
Standard non-bleached
Now, let's create a pre-prompt template:
You are years old male/female. You live in the state of . You come from a low/middle/high-class family. You have [no] children. Please answer the following questions just like a survey respondent would. In giving answers you will strictly follow the required format and will not add any additional words.
And let’s repeat the exercise with a slightly different pre-prompt template that contains the same information:
As a -year-old man/woman from a low/middle/high-class family in with [no] children, respond to these survey questions. You must strictly follow the required format, adding no extra words.
Let’s compare the results:
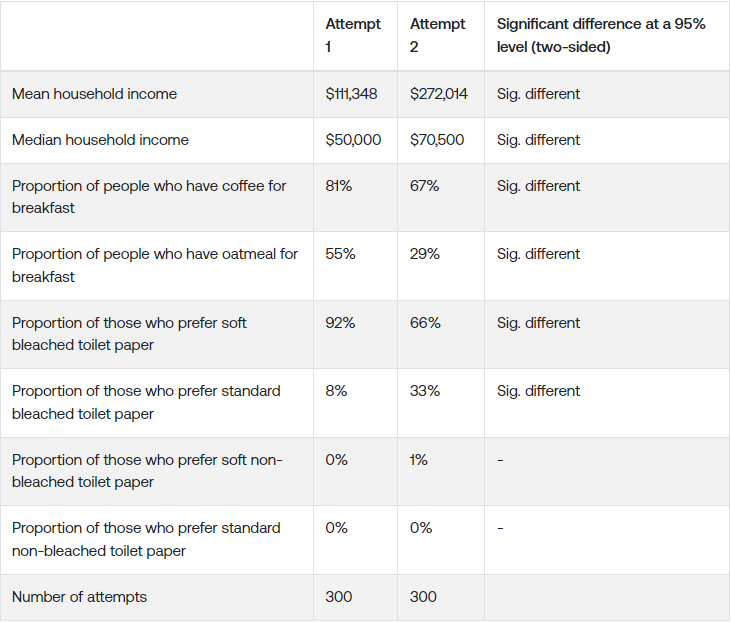
I varied the wording of the pre-prompt (and should note that the randomness parameter was set at 1, which is pretty common and indeed needed to generate different responses for the same demographics), and got completely different results.
To reiterate, the information in the pre-prompt was not different. Just how it was worded massively affected the results, highlighting the low reliability of LLM-generated responses.
Sources of error when using fake respondents
Every research method has sources of error. For survey research, one may list things like sampling error; measurement error; coverage error; non-response error. Here are some of the more peculiar types of errors for fake respondents:
Training data error (i.e. what texts was the LLM trained on?)
Training method error (i.e. what algorithm of training was used?)
RLHF error (i.e. what human feedback was used to adjust the model after the pre-training stage during Reinforcement Learning from Human Feedback? How?)
Prompting error (i.e. what prompt was used?)
Inference parameter error (i.e. what penalties and temperature were set at the inference stage?)
These are plenty of sources of error that are not only novel for most users of survey research, but also incomprehensible because, for example, we do not know what training data was used for GPT-4 or almost any other proprietary model. The same goes for the training and RLHF methods.
Most of the above sources of error would be eliminated if one used real online customer reviews. It’s the same textual format as LLM completions, but without the unnecessary processing and introduction of unpredictable errors during it.
External invalidity of LLM completions
Mark Ritson recently celebrated synthetic data by declaring that “the era of synthetic data is clearly upon us.” He linked a 2022 study about generating perceptual maps from synthetic data that showed remarkably similar results from synthetic data and real respondents for different car brands:
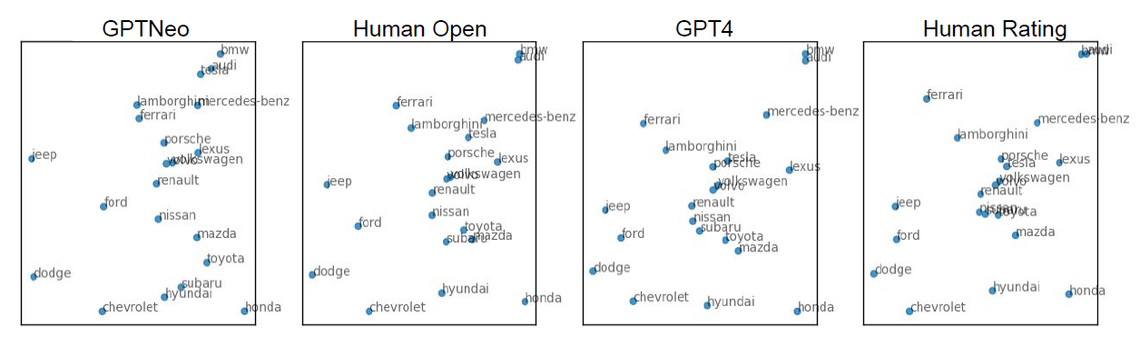
The authors also repeated their analysis on popular apparel brands and reported similarly strong agreement between human respondents and LLM-generated texts.
Why do we see this? Because the training datasets for both GPTNeo and GPT-series models probably contain Americans’ ratings of these popular car and apparel brands. It is not possible to verify this in the case of proprietary OpenAI models because datasheets are kept secret (if they even exist). And it is no longer possible to search the original Pile (the original dataset used to train GPTNeo) because it has been taken down due to copyright violations in at least one of its parts called Books3. But it is entirely plausible that some of the Arxiv articles used to train GPTNeo contained the scoring data related to the car and apparel brands.The authors themselves acknowledge this as a limitation
In our attempt to replicate the analysis from Language Models for Automated Market Research: A New Way to Generate Perceptual Maps" by Peiyao Li et al., we found mixed evidence on the potential for using synthetic LLM-generated data in market research applications like perceptual mapping.
In cases very close to training data distributions, like geography-driven rugby team perceptions, the synthetic data correlated reasonably well compared to human surveys. However, replicating perception maps where there is not a simple heuristic to latch on to appears to be a more difficult task. LLM generated data struggled to capture nuanced associations made by real consumers in less represented domains like Australian café chains.
While some basic clusters are preserved, such as separating out high-end cafés from fast food chains, the relative positioning of brands and inter-brand distances show substantial dissimilarity versus the human survey benchmarks.
Invalidity of sampling frame
The most important reason why we cannot use LLMs for research is that we, as consumers, do not use LLMs to make purchase decisions for us. If we did, then we should be able to survey LLMs instead of people.
Consider wanting to know what baby food mothers want for their children. Normally, you go and survey mothers for that. You do not survey grandmothers with the question, “What do you think young mothers would want for their babies?”
Grandmothers might actually have a good idea of what today's mothers want for their babies. Yet, it’s all too easy to see why the broken telephone effect would make research findings unreliable and largely unusable (unless grandmothers are your true target market).
Similarly with LLMs, unless you can trust an LLM to actually go out and make purchases like a real customer, you should not trust it to accurately tell you about customer behaviour and purchase drivers.
Despite the clear unreliability and invalidity of synthetic respondents, they continue to attract interest from some researchers. In the next article, we will explore the allure of synthetic respondents and why some are drawn to this approach despite its limitations.
For a full list of references and appendices, view the original article here: https://conjointly.com/blog/synthetic-respondents-are-the-homeopathy-of-market-research/