What Kinds of Statistical Tools Are Popular?
There are now many thousands of statistical tools, and new methods are being developed at an increasingly rapid pace
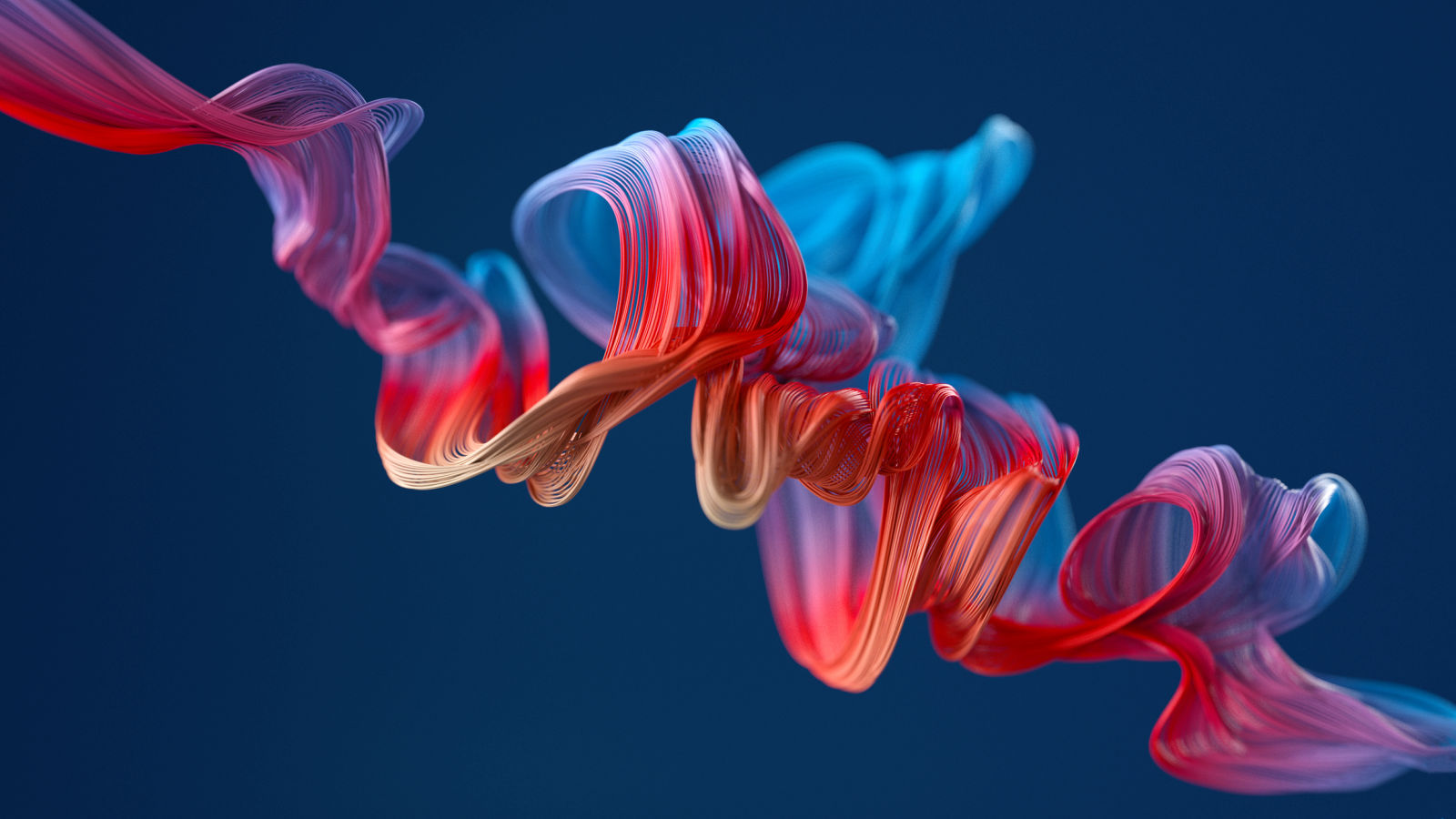
Statistics can be classified in any number of ways, many of which overlap. Most statistical tools belong to multiple categories, as well.
We learn many basic descriptive tools as schoolchildren, for example, frequencies, means, medians, modes and standard deviations. Inferential statistics and hypothesis testing are also covered in many school programs and given a lot of attention in undergraduate "Stats 101" courses. These are used to generalise from a sample to a population and to address the possibility that our results are due to sampling error. If we'd had a different sample, our results might have been quite different, in other words.
Sampling and experimental designs are fundamental to statistics, and associated with them is power analysis which, in a nutshell, is used to help us determine what sample size we'll need for a research project.
Univariate, bivariate and multivariate are other ways to classify statistics. Descriptive statistics such as means are univariate, the popular Pearson product-moment correlation is bivariate, and methods such as multiple regression and factor analysis that make use of three or more variables are examples of multivariate statistics.
Another fundamental way to look at statistical methods is whether they're dependence (supervised) methods or interdependence (unsupervised) methods. Regression, which has a dependent variable, is an example of the first and factor analysis, which does not distinguish between independent and dependent variables, an example of the second.
The type of outcome (dependent variable) is important in regression modeling, in which we attempt to explain or predict one or more outcomes from one or more predictors. We should use different kinds of regression depending on whether our outcome is continuous, binary, nominal, ordinal, count, or time-to-event (e.g., survival analysis). Multinomial logit models are popular in discrete choice modeling and linear regression, for example, would be inappropriate.
There are, at times, heated disagreements - at least among academicians - between statisticians who favour the classical methods most of us are acquainted with and Bayesians. Bayesians look at statistics from a different philosophical angle and have, accordingly, developed a different set of methods. Both approaches are used by practising statisticians, though classical methods are more popular.
Predictive analytics
Much of data science is concerned with predictive analytics - making predictions and classifications. How much a customer will spend in the next year or the likelihood they will subscribe to a new service offered by a company are examples. While this has played an important role in statistics all along, explanation (e.g., causal modeling), in the main, has been more crucial.
Though prediction and explanation are not mutually exclusive, very different skill sets and, frankly, mindsets are required. In explanatory modeling, subject matter knowledge is critical as is being able to interpret our model. Samples sizes may be very small, and normally there is no need to develop a predictive algorithm. Many techniques less well-known in data science, such as mediation and path analysis, are utilised.
Related to this point are parametric, semi-parametric and nonparametric methods. Many prediction and classification algorithms - often called machine learners - are considered nonparametric statistics by statisticians. Put simply, nonparametric methods make fewer distributional assumptions and are more data-driven than parametric methods. Semi-parametric statistics fall in between the two.
We also should distinguish among methods intended for cross-sectional, longitudinal and time-series data. Cross-sectional data, representing one slice in time, have historically been most common in many fields. The distinction between longitudinal and time-series data is frequently unclear, but both refer to data collected over time. Generally speaking, longitudinal models have few time periods (e.g., 6-8), while time-series analysis is used when there are many periods (e.g., 50 or more). The statistical methods used for these three sorts of data are quite different.
Spatial statistics, spatiotemporal modeling and time-varying coefficients are other areas which receive a great deal of attention in some disciplines. For example, we might wish to examine national and regional sales of our product over time with a single model. Certain predictor variables may be helpful in understanding and forecasting trends and other patterns in sales. The influence of these variables on sales may vary over time, and various methods have been developed to account for this.
Functional data analysis, nonlinear statistics and assorted techniques intended for data where relationships between predictors and outcomes are not straight-line are of great interest in many disciplines. Generalised Additive Models are one kind. These are complicated subjects and I'll just say here that the occasional contention that statistics is only appropriate for "linear" relationships and normally distributed data is badly mistaken.
Social network analysis is a highly complex area of statistics important in sociology, marketing and several areas of data science. Defined dryly by Wikipedia as "the process of investigating social structures through the use of networks and graph theory," these methods have attracted considerable attention in recent years.
Multilevel and multigroup modeling
Multilevel and multigroup modeling are occasionally confused by non-statisticians. They are quite different but, in some circumstances, can be combined. Beginning with the first, data may be hierarchically structured - customers within bank branches within regions, for instance. If we ignore this structure, our parameter estimates (e.g., regression coefficients) will be less precise.
In multigroup modeling, data from different groups are combined and modeled simultaneously. This is a common technique in psychometrics for assessing measurement invariance. It has a similar role in marketing research. For example, we may wish to examine if attitudinal items in a consumer survey are interpreted the same way in different consumer groups. Marketing researchers often take this for granted. However, in some kinds of research - multinational and multicultural studies, in particular - making this assumption can be risky.
Factor and cluster analysis are familiar latent variable methods. In the first, the latent variables (factors) are continuous, and in the second, they (the clusters) are categorical. Mixture modeling is a sophisticated extension of this able to combine the two, for example, in factor mixture modeling. Mixture modeling is also used with regression, for instance, when we suspect different latent classes (clusters) have different priorities.
How to deal with missing data is an important and often contentious area of statistics, and many methods have been developed. This is a very big topic.
http://cannongray.com/methods may be of interest to those looking for books and other resources on these topics.
There are now many thousands of statistical tools, and new methods are being developed at an increasingly rapid pace. Existing methods are being refined and extended in addition. In light of this, I hope you've found this snapshot helpful!
Kevin Gray
President at Cannon GrayCannon Gray was established in 2008 by Kevin Gray, a marketing scientist who has been in marketing research for more than 30 years. Previously Kevin had worked for The Nielsen Company's Consumer Research division, Kantar Research International and McCann Erickson as well as on the client side.
His background covers dozens of product and service categories and over 50 countries. A member of the American Marketing Association and the American Statistical Association, he is always keen to learn about innovations in marketing research and to borrow ideas from other disciplines.