Rewriting the Rules of Concept Testing: How AI is Powering Smarter Product Decisions
AdvertorialIn a rapidly changing market, brands can't rely on intuition and outdated benchmarks. Many teams still depend on traditional KPIs and extensive manual analysis for concept testing.
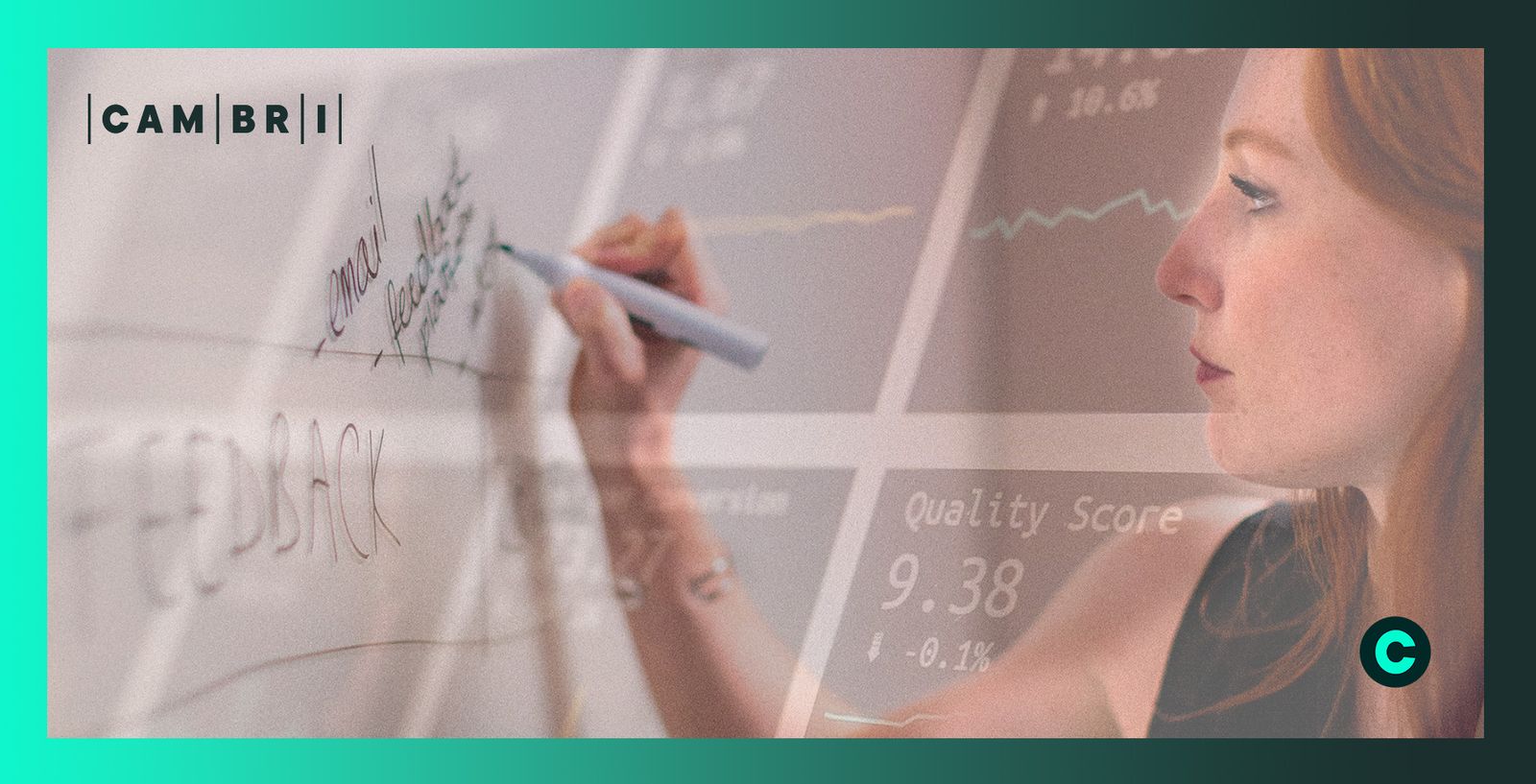
In a world where consumer expectations evolve overnight and innovation cycles are accelerating, brands can no longer afford to rely on gut feel and static benchmarks to guide product development. Yet for many teams, concept testing still hinges on traditional KPIs, like purchase intent or uniqueness, supported by hours of manual analysis of open-ended feedback.
The process is slow. The outcomes are often subjective. And more importantly, it doesn’t scale.
At Cambri, we believe it’s time for a smarter, faster, and more consistent approach. That’s why we’ve built an AI-powered platform that helps brands not only predict product success but also understand exactly how to refine concepts to make them stronger, turning thousands of raw consumer opinions into clear, data-driven decisions.
Why Traditional Concept Testing Falls Short
Historically, concept testing has followed a familiar formula: scorecards filled with quantitative KPIs supported by a qualitative read from open-ended responses. Insights professionals would pore over hundreds, if not thousands, of comments, using their expertise to interpret what consumers were really saying.
The problem? This method is deeply reliant on human interpretation, making it time-consuming, inconsistent, and prone to confirmation bias. Even when open-ended feedback is rich with consumer insight, it often gets reduced to word clouds or anecdotal examples.
More critically, open-ended responses have rarely been used to drive the decision-making process. Instead, they’ve served as supporting colour around KPI trends—rather than being a source of predictive power in their own right.
“Including open-ended data in the prediction model boosts accuracy by up to 30%—turning raw feedback into real foresight.”
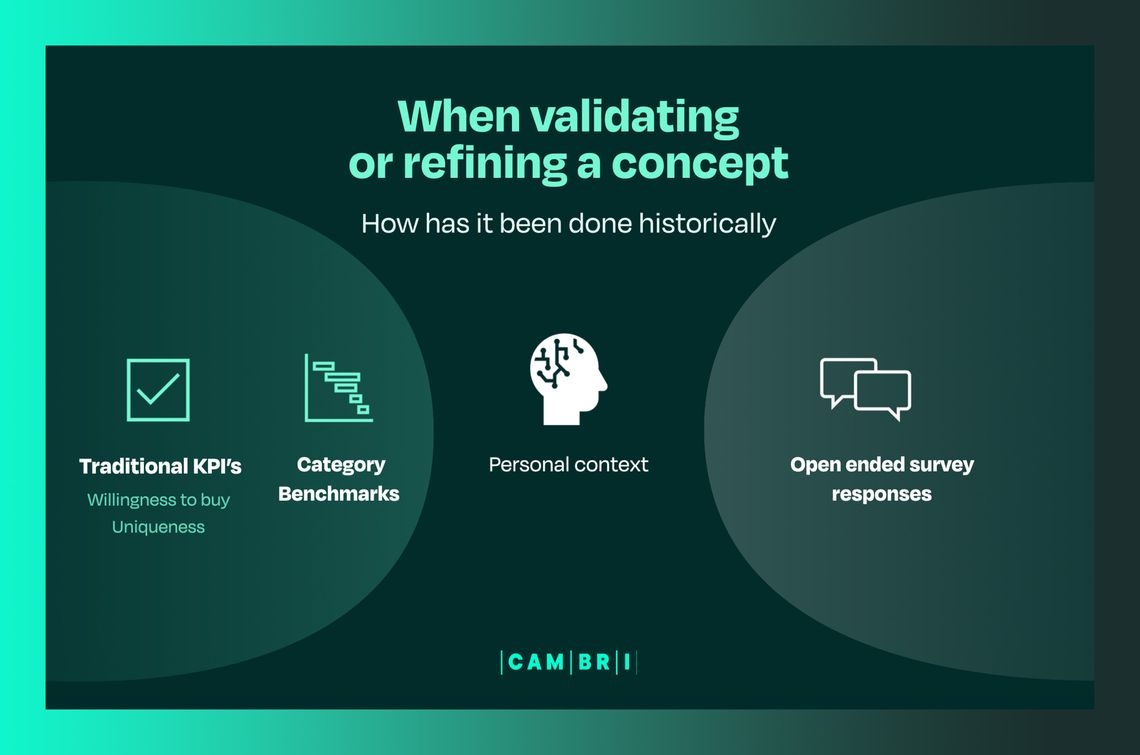
Unlocking the Full Value of Open-Ended Feedback
Our work at Cambri shows that it doesn’t have to be this way. In fact, when AI is used to integrate open-ended feedback into a predictive framework, the accuracy of product success forecasts increases by as much as 30%.
That’s because AI, specifically Natural Language Processing (NLP) and machine learning, can process vast amounts of qualitative data, identify patterns, understand sentiment, and quantify the relative importance of different themes. It does this systematically, consistently, and in real time.
We’ve trained our models on over a million open-ended responses, creating a structured taxonomy of drivers grounded in academic research. These AI models don’t just summarise the feedback—they translate it into actionable insights and measurable impact.
From Data to Direction: How AI Refines Product Concepts
Let’s say a brand is testing a new plant-based noodle concept. Our AI platform assigns it a Launch AI Score, a predictive measure of likely market success based on a combination of KPIs, in-market performance and holistic survey data plus qualitative themes.
But the real power lies in what happens next.
The AI reveals which elements of the concept are driving that score—both positively and negatively. For example, consumers may be excited about the protein content and packaging convenience (positives), while hesitant about flavour combinations or pricing perceptions (negatives). Each of these drivers is quantified, so the team can see exactly how much they contribute to the overall score—and where improvements will have the biggest impact.
Teams can then drill down into the individual consumer comments that support each theme, ensuring full transparency and traceability.
The result? A clear roadmap for concept refinement, backed by robust data—not guesswork.
“AI doesn’t just summarise what consumers say—it shows you exactly what to do next.”
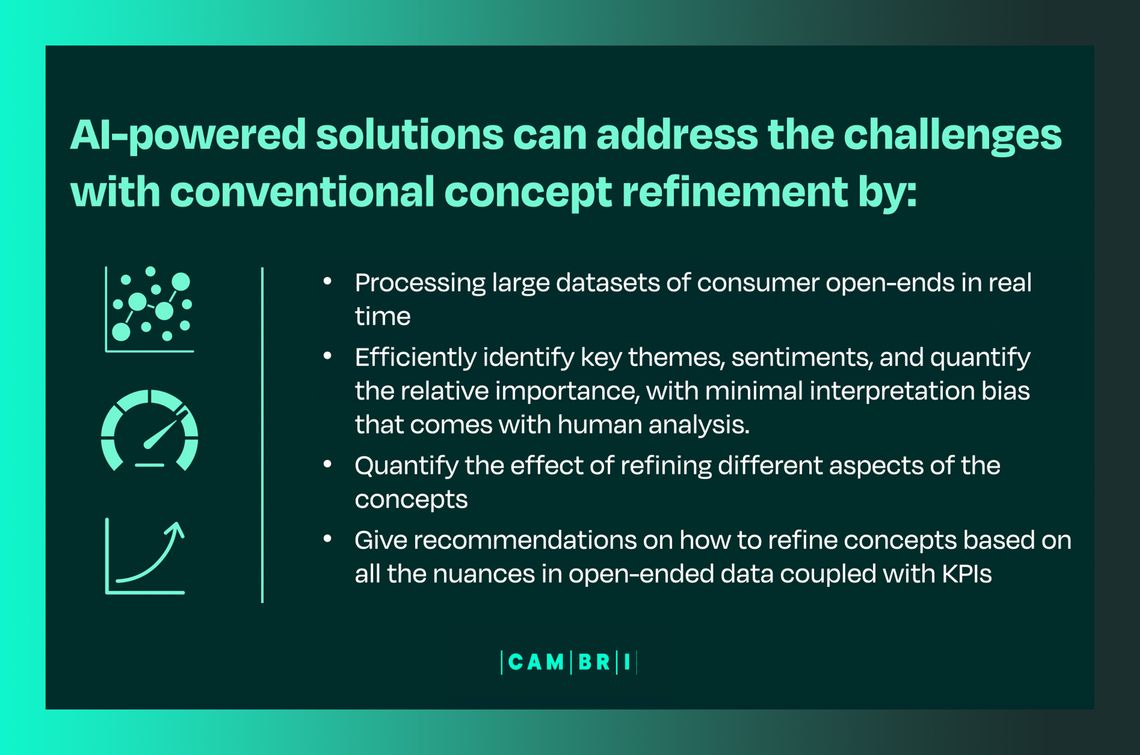
Why This Matters for Innovation Teams
AI isn’t just faster, it’s transformational. In internal comparisons, our AI-driven analysis has proven to be up to 20 times faster than traditional methods. This dramatically increases testing capacity, shortens innovation cycles, and enables more iterative, agile ways of working.
Equally important is consistency. Because the analysis is systematic and model-driven, it eliminates the variation that comes from having different individuals interpret feedback in different ways. This allows large organisations to scale insights across teams, geographies, and product categories without compromising on quality.
Tackling Common Challenges—From Bias to Sarcasm
What about bias in consumer responses, or the occasional sarcastic remark that can throw off sentiment analysis? Our NLP models are built to handle this. By averaging across large datasets and training the model to identify sentiment and intent in context, we reduce the impact of outliers and filter for the signals that truly matter.
The result is a more accurate, more objective view of how consumers perceive your concept—and how to make it better.
A Smarter Way Forward
“With AI-powered analysis, we’ve seen a 20x increase in speed compared to traditional concept testing methods.”
At Cambri, we see AI as a partner for innovation. It’s not here to replace human judgment, but to enhance it—providing clarity, speed, and structure where it’s needed most. By combining classic KPIs with sophisticated analysis of open-ended feedback, we’re helping brands move from intuition to evidence, and from idea to in-market success.
If your team is still spending hours dissecting word clouds and debating interpretations, it may be time to ask: is there a different way?
We believe there is. And it starts with AI.
Discover how AI can supercharge your concept refinement — download the free eBook at cambri.io/resources/ai-concept-refinement?RWQ12025
Thomas Berthelsen
Head of Managed Services at CambriThomas Berthelsen is the Head of Managed Services at Cambri, delivering projects that provide insights at every stage of the innovation process. He helps brands refine concepts, optimize pricing, validate innovation, forecast success, benchmark performance, improve go-to-market communication, optimize portfolios, and identify white space opportunities. With deep expertise in quantitative and qualitative research methodologies, his team executes full-service research projects using Cambri’s AI-powered concept testing platform. At Cambri, Thomas ensures clients benefit from robust research frameworks, predictive modelling, and AI-enhanced methodologies, enabling them to generate actionable insights, refine concepts with confidence, and launch more successful products.