Unleashing AI's power in quantitative analysis
"A few clients mentioned their successful large-scale tests enabling them to produce far more sophisticated market segmentation analyses. "
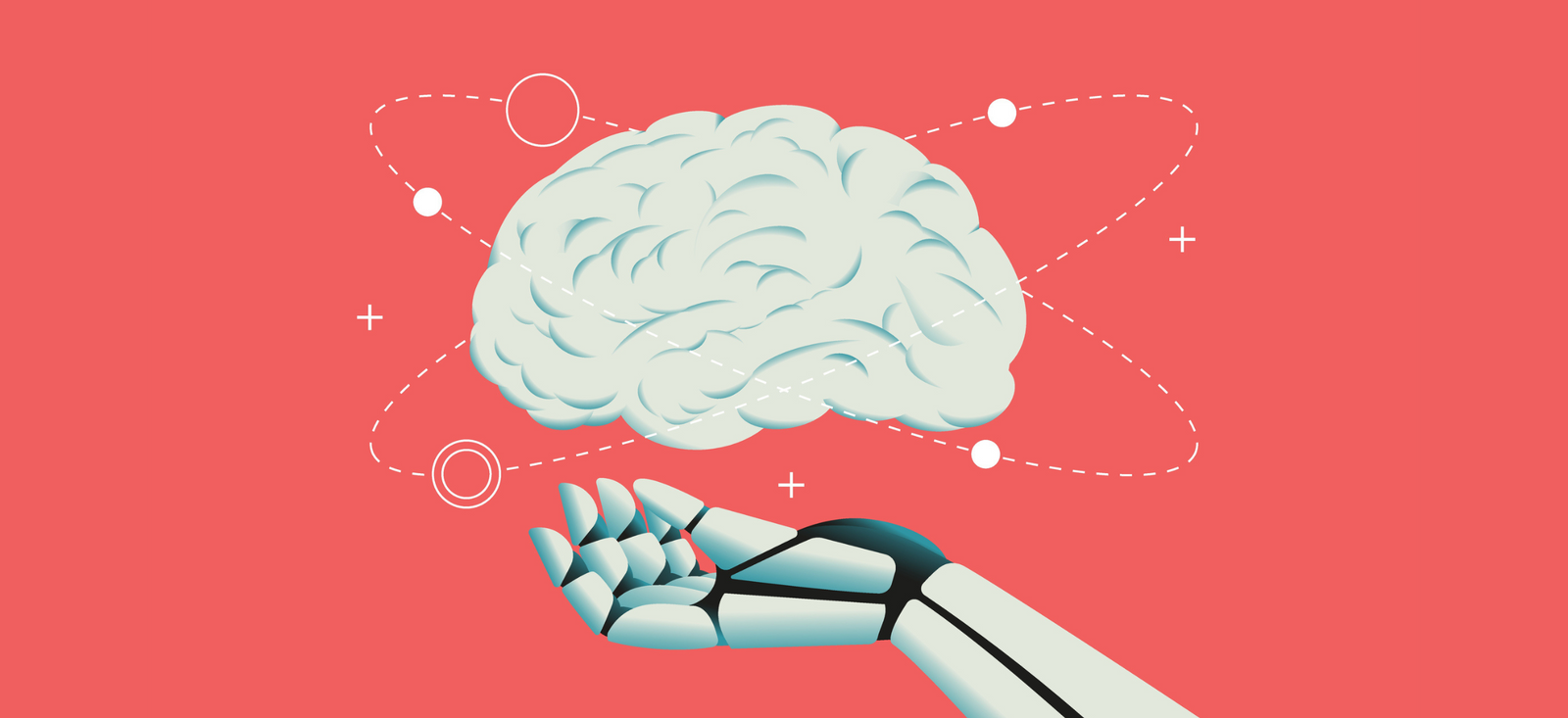
Article series
AI Taskforce Roundtables
- The evolution of research methods
- Enriching qualitative insights
- Unleashing AI's power in quantitative analysis
- Crafting compelling narratives from AI-generated Insights
- Adventure Table - GPT Exercise: Practical AI Engagement with ChatGPT
- Pros & cons: The dual faces of AI
- Bias and ethics of Artificial Intelligence
Using LLM for text analytics
There seems to be a widespread interest and adoption of using LLMs for the summarisation and open-ended analysis, opening up the opportunity to change how research conducts research, a blending of quant with qual. Its value seems to be pretty universally recognised.
The primary constraint for Chat GPT appears simply its token cost, which can escalate quickly when used extensively.
As noted by Ipsos, while LLMs perform well in English, results in non-English languages can be inconsistent, with some not yet adequate for practical use.
Synthetic data creation
There was extensive dialogue about using AI to create synthetic data. The main use case scenarios cited were to help reduce sample sizes, fill in data gaps, and shorten surveys for respondents. But also to do more robust analysis (i.e. scaling up data, not cutting back). There was an acceptance that training data sets to produce synthetic data needed to be robust.
A few clients mentioned their successful large-scale tests enabling them to produce far more sophisticated market segmentation analyses.
However, some voiced concerns about its current practicality, emphasising the challenges of producing reliable synthetic data. Proper data formatting and thorough cleaning prior to analysis are crucial, making it a specialist solution for now.
A critical takeaway: AI can't perform statistically impossible feats. Expanding 100 responses to 1,000 answers goes beyond basic statistical extrapolation's limits.
Training data
The possibility of creating data lakes and pooling all the research data a company has into one pot was discussed to produce training data to prime LLM for generalise analysis.
Whilst a number of people have expressed interest in doing or having access to specialist research data sets that could do this, none other than Ipsos actually seem to be doing anything in this area just yet.
Presently, token volume limitations appear to be the most significant hurdle to test and experiment.
The insights from IPSOS highlighted the vast amounts of training data needed to produce meaningful, reliable analysis. Considering that LLMs are trained on trillions of words, uploading a mere few hundred survey responses is insufficient.
Using LLMs to create a persona and generate synthetic research answers.
This was a big topic of conversation; a number of companies appear to be actively exploring the abilities of LLMs to conduct pseudo-research and test out their capabilities to do this. Most conclude that right now, that is an interesting opportunity, but they don’t trust it.
This reinforced the message from the Human8 presentation, which focused on the results of an experiment where they compared the answers from a community of real respondents who were asking questions about their views on certain car brands to some pseudo answers from LLM-created personas. The results, on the surface, were impressive but appeared to lack the authenticity of real responses.
Conclusion:
LLM is good for discovery and ideation, but anything it suggests needs validation with real research.
Using AI for ideation and new product development
At the event IPSOS revealed they have already launched a solution using LLM’s to develop new concept and product ideas. In my discussion groups, there was interest but none had explored this yet.
Prompt libraries
Much was talked about the emerging skills of writing prompt to effectively gather research intel. A sense that this was an emerging skill that everyone wanted to get on top of.
There was a request/desire for a training to learn best how to use LLM for certain common research tasks.
Using AI to aid in the analysis of data
A couple of people in the discussions we had talked about how they were experimenting using LLM to analyse data using agent tools. One person talked about an experiment they had conducted where they fed some sequel queries into an LLM which worked with an agent tool to interrogate some tracking data, enabling them to interrogate it using simple questions. e.g. “tell us how our brand is doing compared to other in the last quarter”. They said they were impressed with how well it worked, but it took a lot of effort to get the data set up and properly tagged the data, placing limitations on how easily it could be used, but they were very excited about it.
This does sound like a very interesting idea, that was welcomed by the group discussing this who all could imagine a world free from excel spreadsheets.
Using AI for smarter data visualization
Similarly one person mentioned they were using llms to help them with data visualisation tasks – asking it to write python code to access R and create charts and data vis. There was some excitement about the all round possibilities in this area – how it could be used to make everyones lives simpler and reduce reliance on powerpoint.
Expected future uses of AI for market research
To shorten surveys
To reduce sample requirements or to augment the sample
Aid in the scripting and crafting of surveys
Smarter analysis of quant data– less technical, less Excel more question-based interrogation of data
To help jump-start research projects – get out ideas onto the table and create a hypothesis quickly from which real research can be used to investigate.
Closing the gap between qual and quant – envisaging a hybrid future
Helping with cleaning, organising and cataloguing data
Bringing a different perspective on data and discovering hidden incites
Helping with the storytelling and bringing data to life by connecting it with generative AI visualisation tools
Replacing Excel to enable language-based data analysis prompting –“talking to data in the human language.”
Improvement in all round efficiencies of everyday work practices
Broader hopes and dreams for AI in research
To make research more fun and exciting by making things simpler and easy – not having to be a tech wizz to analyse data.
General Concerns about AI
Sample source bias of the training material used to prime LL M’s – white, western, male perspective and English language dominance.
Lack of transparency on source of training data and not being able to quantify the biases in training data (an opportunity for someone to devise a good technique to do this)
Over confidence of LLM’s - producing plausible sounding incites that are off.
“Inbreeding” The circular loop of AI being fed by its own AI generated content will start to corrupt things (apparently there are already now more AI generated images on the web that real one)
Regression to the norm – every reaching the same conclusions, lack of novelty.
Loss of freshness – training data being lowest common denominator the creation of an “idiocracy”
The feeling that it will make us more stupid less novel
Concerns that research companies were voicing:
Client going directly to solution providers, bi-passing research companies
Lack of technical skills and resources to develop competitive solutions
Summary observation
General fear around “not being left behind” with AI and FOMO on what everyone else is doing. Huge appetite for the intelligence of what everyone is doing. Sense that people also keen to learn and reset their approach.
Jon Puleston
Vice-President Innovation Profiles Division at KantarJon Puleston serves as Vice President of Innovation for Kantar's Profiles Division. In this role, he heads QuestionArts, an international team specializing in the design of surveys and the development of specialist tools and technology for conducting research in the online and mobile arena. Additionally, he serves as a consultant on survey design best practice to companies around the world.
Over the last few years he and his team have won multiple awards for their ground breaking research on research exploring survey design methodology and in particular for their work in the field of gamification of research, survey optimization and prediction science.
Article series
AI Taskforce Roundtables
- The evolution of research methods
- Enriching qualitative insights
- Unleashing AI's power in quantitative analysis
- Crafting compelling narratives from AI-generated Insights
- Adventure Table - GPT Exercise: Practical AI Engagement with ChatGPT
- Pros & cons: The dual faces of AI
- Bias and ethics of Artificial Intelligence