The allure of synthetic respondents - Part Three
Part three in a three-part series that explores synthetic respondents and their impact on the market research industry.
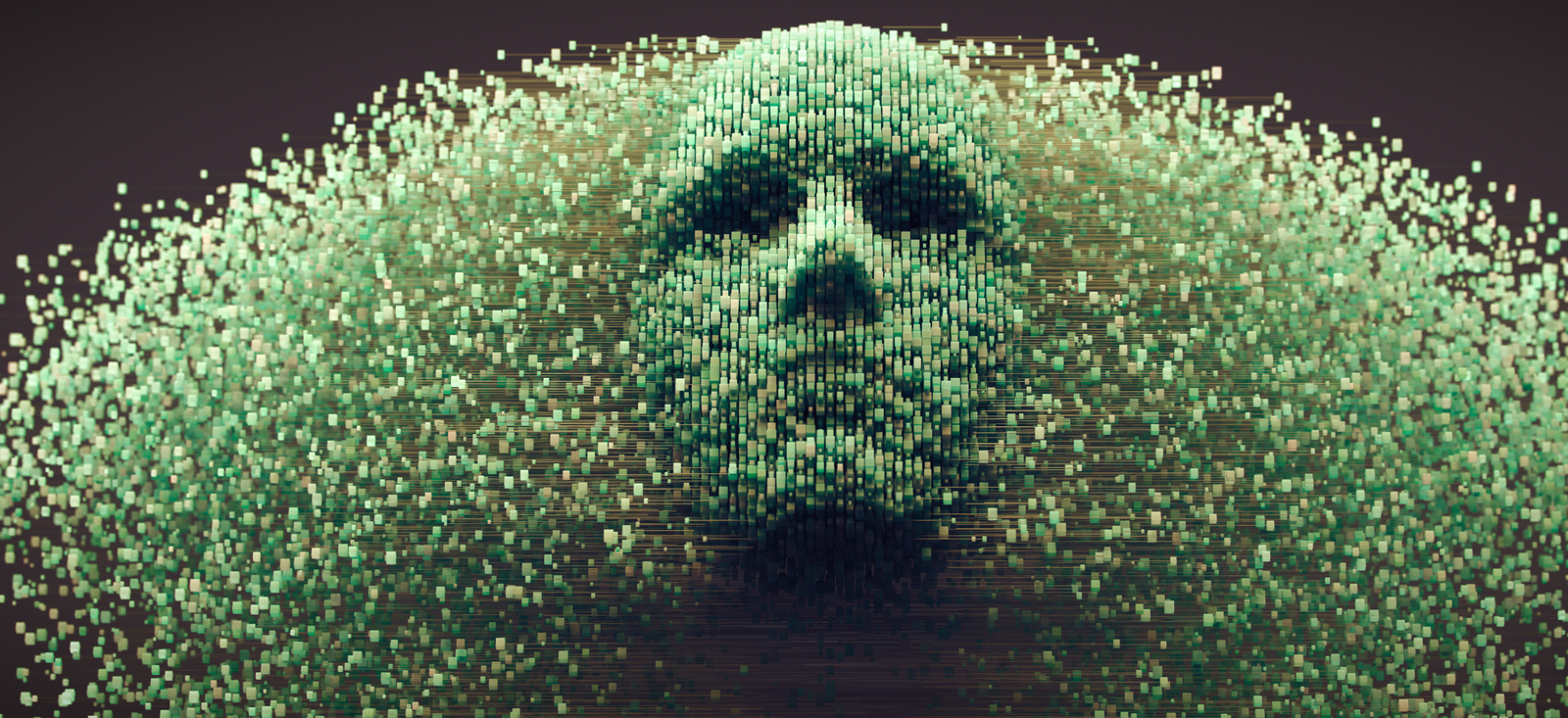
Despite the unreliability of synthetic respondents, their appeal persists due to promises of speed, cost savings, and reach.
In the first two articles in this series (article one here and article two here), I have explained what synthetic respondents are, and why they produce unreliable results. So why would anyone still consider using them?
The allure of synthetic respondents comes from several factors:
faster execution,
the promise of lower costs,
the promise to reach hard to get audiences,
the ability to ask questions that would be difficult to pose to real people.
While these benefits may seem attractive, they are akin to the promises made by séances as a market research method. Both synthetic respondents and séances fail to provide reliable answers to business questions.
Playing hard make-believe
LLM responses themselves are designed to exploit gullibility because they are meant to produce human-like text through two main processes:
Pre-training. This is an unsupervised learning step (unsupervised in the sense that there is no human who gives a model "sticks" or "carrots"), in which the systems ingest a lot of scraped data. This typically includes copyrighted texts — without authors' consent, credit, or compensation. The model learns to mimic human text, which already has many sentences like "Burgers are tasty", "Charity is good". So even after this step if you could prompt the model with "Burgers are " and it would likely auto-complete to "tasty".
Reinforcement learning from human feedback. This is a somewhat complicated step, but it relies on a quite basic activity. A large group of people (often underpaid in countries where labour laws are not strong) sit in front of a computer all day and are exposed to various completions from the first step. Their job is to press 👎 or 👍. Based on such "sticks" or "carrots", the model is moulded into something that even better represents human answers, not necessarily truthful answers, often sycophantic ones instead.
So when someone makes an observation that there are correlations between an average human answer and an LLM completion, it is not at all surprising, interesting, or note-worthy. That's what LLMs are designed to do.
On top of that, the economics of a synthetic data provider is quite favourable compared to a market research firm. They do not have to spend money on sample, the hard labour of the fieldwork team who check response quality, and they can also churn projects quicker. That means they have more money left on sales. Therefore, expect them to deliver very slick presentations.
An unstable phenomenon
If you have been keeping an eye on the fake data market over the past year, you may have noticed the language used to promote synthetic respondents has slowly changed. Some sellers first hid the use of synthetic data, then proudly displayed it, and now prefer flowery terms like “augmented audiences”. From lack of disclosure we moved to “LLM-generated responses” to “data based on or including LLM-generated responses”, but without full disclosure of methodology.
If it is no longer about generating answers using LLMs, what is it? What is the secret sauce for this dish? Why not explain it widely? My most parsimonious hypothesis is a lack of a solid, stable methodology that they would be comfortable sharing publicly.
There has not been any other wave of innovation in the research industry where methodology is not widely reported and understood. In most cases in the past, research methods came from academia after plentiful reviews. One famous exception, of course, is the Price Sensitivity Meter, which was introduced in this publication in 1976, but with a great deal of detail.
If the validity and reliability checks I show above are not relevant to this new “data based on or including LLM-generated responses”, then it is incumbent upon sellers to show what is.
Let’s also get clear answers:
How exactly are sources, including traditional survey data, publicly available statistics, trend reports blended into synthetic data? If at all?
How is “real-time data synthesis” performed?
How are novel terms like “knowledge lake” and “data amalgamation” precisely defined?
What are the differences between “virtual audiences”, “augmented data” and “synthetic data”?
The answer might be that this newer breed of synthetic data is just putting a bunch of information about respondents like raw online reviews or existing research reports in the pre-prompt for an LLM. But if your synthetic data provider simply repackages existing data like online reviews, why not use the original data directly without their help?
When can you actually use synthetic data?
I hope I convinced you that you cannot use LLMs for real business questions. What is synthetic data useful for? There may be some cases:
Testing questionnaires. In this case, it should be called “questionnaire testing using LLM-generated responses” (not “market research”) and you would need to write a scrappy JavaScript at the cost of a few hundred dollars, not hire a platform company to do it.
Early-stage idea generation. In this case, again, no need for a synthetic data company at all. Anyone will do just fine with Chat-GPT.
Quality assurance in UX / UI contexts. For example, the Commonwealth Bank of Australia has an ongoing project to pre-test messages and simulate behaviour given various UI changes without ending the use of real customers’ feedback and research responses.
When you need a placebo effect from a project, and do not care about the answers.
The last case is very interesting. I have seen more than one research or consulting project that was done for the sake of having a project. The management may be completely disinterested in the outputs, but, for whatever reason, still assigns a team to do something. If you are doing a placebo project and do not want to do surveys, consider doing secondary or tertiary research instead. Read what others have written about your topic. If you like talking to people, try a focus group, or use the research budget to hire a freelancer to do the research for you.
Where does the fake data trend take us?
The somewhat uncritical reception of fake data companies in the industry press as well as industry bodies poses an awkward question: Do we as an industry allow just about anyone among our ranks? Will any methodology do, or should we demand more proof for new techniques?
As for companies who supply fake responses and declare that to be the future of market research? I suspect they will continue to exist, just as homoeopathy still exists.
Caveat emptor.
For a full list of references and appendices, view the original article here: https://conjointly.com/blog/synthetic-respondents-are-the-homeopathy-of-market-research/