Behavioural economics
Behavioural science in quantitative research: Part two
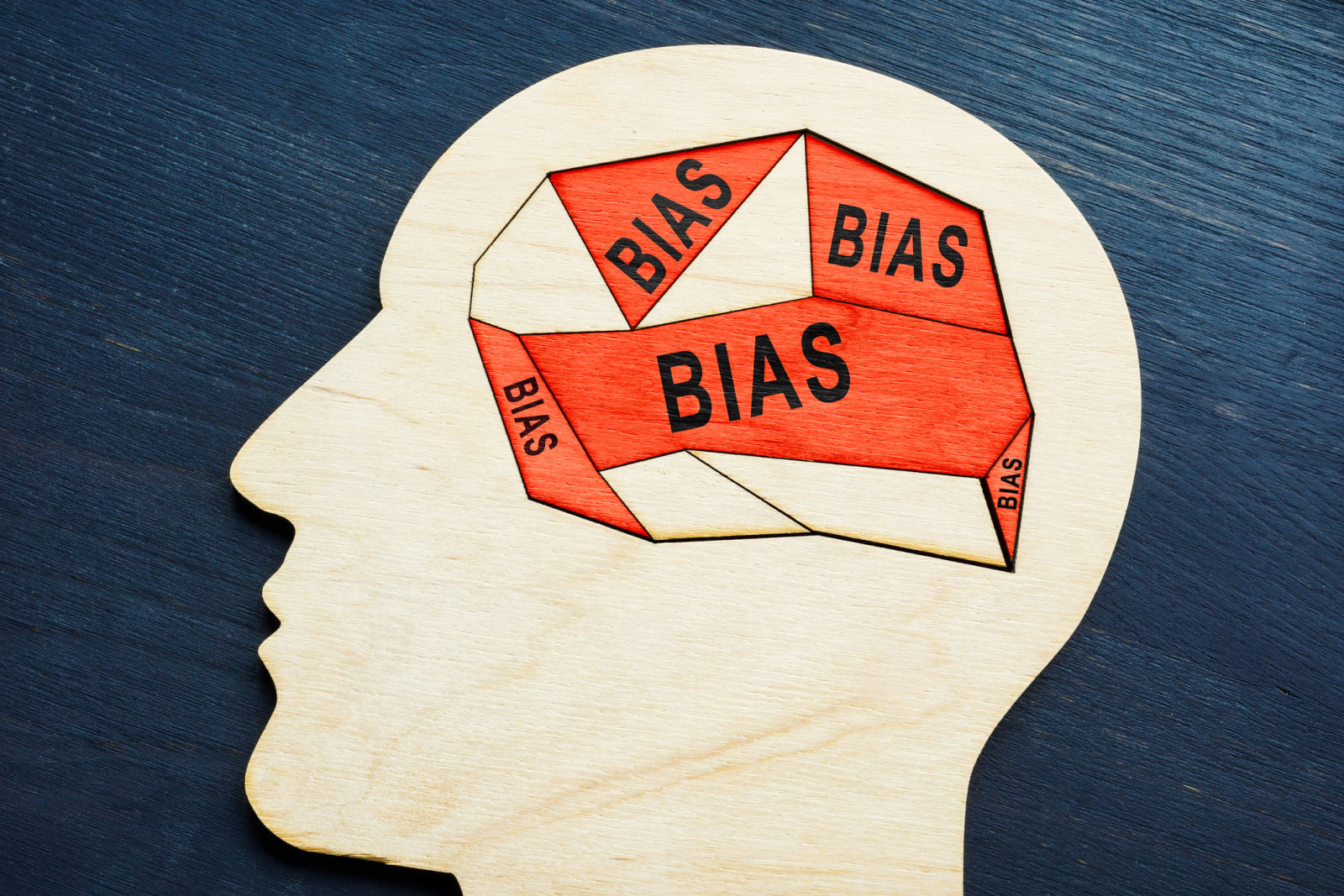
Article series
Behavioural Science in Quantitative Research
- Behaviour change models
- Behavioural economics
- Personality psychology
As was discussed in part one of this three-part series, a huge opportunity exists for clients to improve their ability to understand, predict, and influence key stakeholder behaviour – through applying behavioural science insights and approaches specifically to quantitative market research. However, as was also discussed, behavioural science presents two key challenges, both to quantitative and qualitative research.
First, human decision-making and behaviour has been shown to often be complex, surprising, and even seemingly illogical.
1. Therefore, how do we best understand human complexity, and minimize the chances of making incorrect assumptions?
Second, human decision-making and behaviour has been shown to be influenced by a range of subtle factors, of which research participants may be unaware.
2. Therefore, how do we fully unravel this complexity if there are some things research participants can’t tell us?
Following on from part one of this series, which focused on behaviour change models, this article considers behavioural economic approaches. In response to the challenges above, it first explains how such approaches can help facilitate a deep understanding of human decision-making and behaviour. Second, it demonstrates how, when employed quantitatively in particular, they can help unearth important influences of which participants may be unaware.
Behavioural economics
Similarly to behavioural science (BS), behavioural economics (BE) is fundamentally about understanding why we do what we do. However, BE is somewhat narrower in scope, focusing typically on economic behaviours. In terms of the first key challenge above, BE has played a particularly large role in drawing attention to the complexity of human behaviour (as well as often the more illogical aspects). However, as well as laying down challenges, those working in BE have provided the language to help others make sense of those more complex and surprising observations.
The first of two important BE concepts is the heuristic. A heuristic is a shortcut “rule of thumb”, typically used to help decision-making in more cognitively challenging situations. Heuristics often work well, however they can sometimes go wrong. In particular, heuristics are responsible for a range of what are known as cognitive biases. These describe systematic errors in thinking. Three well-known cognitive biases are discussed below for illustrative purposes.
Many qualitative researchers have adopted the language of heuristics and biases. With, in particular, many dozens of cognitive biases identified and documented by behavioural economists, a significant opportunity to derive deeper insights from qualitative research has been embraced by many.
Quantitative application – Basic
In quantitative research, heuristics and biases can also be identified. To illustrate, risk aversion is a bias whereby in economic decision-making, people prefer outcomes with low uncertainty to those with high uncertainty – even if this means giving up the chance for potentially greater rewards. Risk aversion can be identified quantitatively through a simple, direct question. For example, in a study with 100 physicians we asked: “How would you describe your approach to trying new treatments, using the following 7-pt scale?”.
The responses showed more than half of physicians (based on “top 3 box” agreement scores) said they prefer to wait a while before trying new treatment approaches (52%). In contrast, only just over a quarter (28%) said they are among the first to try them. These findings confirm a high incidence in this population of risk aversion. This general tendency to avoid uncertainty will likely present a key challenge to any provider trying to encourage uptake of new treatments in the future.
A problem
However, while cognitive biases such as risk aversion may be widespread, a significant limitation is quickly encountered with this approach. As discussed above in the second key challenge, research participants’ decision-making and behaviour is often influenced by subtle factors of which they are unaware. Unfortunately, many cognitive biases (and heuristics) fall into this bracket.
One illustration comes from a study in the US, where doctors recommended significantly less pain medication for black or Latino patients than for white patients with the same injury. The authors attributed this to unconscious bias. This is where people make decisions based on the personal characteristics of others – without conscious awareness.
If there are some things research participants can’t tell us, direct quantitative questions to identify biases can therefore only take us so far. Similarly, in a typical qualitative setting, the extent to which the full range of biases can be reliably uncovered is limited by the information participants are able to divulge. However, in quantitative research, methods exist to help tackle this challenge.
Quantitative application – Detailed
The most effective way to explore hidden drivers quantitatively is through a randomised controlled trials (or split sample) approach. To demonstrate we can examine one final bias: over-confidence. This is observed when people’s subjective confidence in their ability is greater than their actual performance. However, similarly to unconscious bias, those afflicted are unlikely be aware of this tendency.
To illustrate over-confidence, we asked half (n=50) of a physician sample to estimate the percentage of their own practice antibiotic prescriptions they felt to be justified. The other half (n=50) of the quantitative sample were asked the same question – but instead about their own personal behaviour. The results demonstrated a clear, statistically significant difference: 80% of physicians’ own personal prescriptions were thought to be justified – while the figure for own practice was just 58%. Especially when combined with high levels of risk aversion (see above), over-confidence therefore presents a key challenge to providers encouraging uptake of new treatments.
Conclusion
BS is clearly highly relevant to MR. However, as discussed it presents two significant challenges. In response, when used quantitatively in particular, BE methods can help us uncover a wide range of behavioural influences – whether research participants are aware of them or not.
Look out for the next article in the series, which will explain how personality traits can be used to help clients better understand, predict, and influence key stakeholder behaviour.
This article series contains selected highlights from “Applications of Behavioural Science to Quantitative Market Research” – a new 35-page guide written by Activate Research which will be released on 27th September 2022. To pre-order a free copy
Chris Harvey
Founder at Activate ResearchChris Harvey is the Founder of Activate Research. He is an expert in helping research agencies add complementary insights from behavioural science (and psychology more broadly) to their research offer, enabling end clients to better understand, predict and influence target audience behaviour. Chris has over 10 years’ experience in the research industry, working for agencies including Dunnhumby, GfK and YouGov, and in 2016 achieved a Distinction in MSc Behavioural Science.
Article series
Behavioural Science in Quantitative Research
- Behaviour change models
- Behavioural economics
- Personality psychology