Innovation in statistics
Happily, a lot has happened in the field of statistics since the Roaring Twenties, when writers such as Dashiell Hammett and Ernest Hemmingway were getting their careers underway.
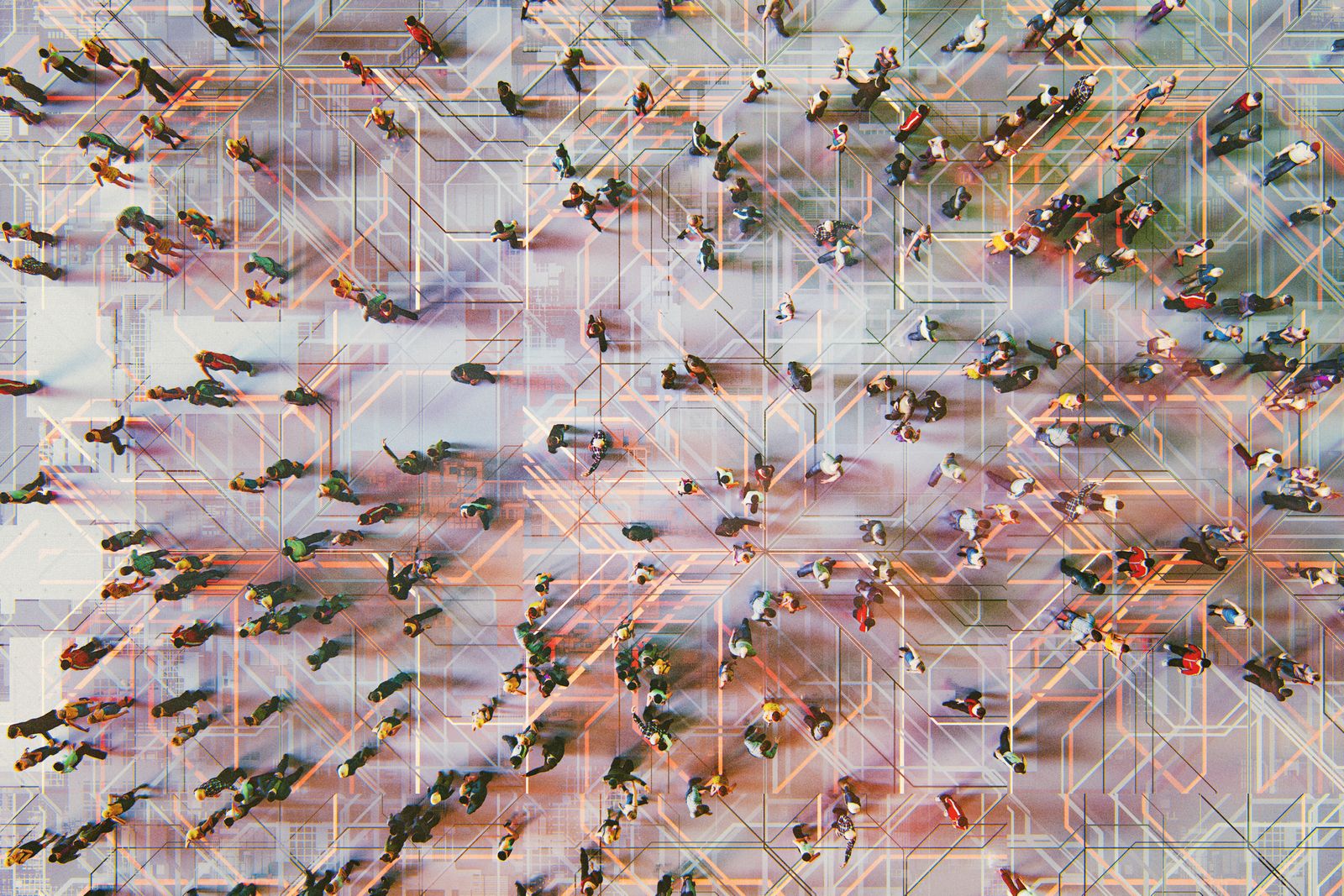
Many people associate statistics with means, standard deviations, t-tests, correlations and other topics covered in “Stats 101” college classes. In these classes, we’re presented with a catalogue of formulas and examples of how to use them to accomplish specific (and often uninteresting) tasks. All these methods and formulas are at least a century old, and, in the main, introductory stats classes are pretty dull. I view them as I do stretching and warmup exercises - essential but not thrilling.
Happily, a lot has happened in the field of statistics since the Roaring Twenties, when writers such as Dashiell Hammett and Ernest Hemmingway were getting their careers underway. Quite a lot of recent innovation in statistics has been driven by the needs of researchers working in finance, genomics, neuroscience, epidemiology and ecology, though all fields have been affected in some way by these new developments. Websites for the Royal Statistical Society and the American Statistical Association provide a glimpse of the latest innovations in statistics.
Nonparametric statistics is one area where there have been many new developments. Put simply, these are methods that make few assumptions regarding our data or model. They are more data-driven than parametric methods. The Wilcoxon rank sum test and Spearman’s rank correlation coefficient are two venerable nonparametric techniques some of you may recall. The Oxford Handbook of Applied Nonparametric Econometrics (Racine et al.) may be of interest to the technically inclined.
Machine learning is statistics unless it’s used for purposes other than analysing data. Popular machine learning approaches such as bagging (e.g., random forests), boosting (e.g., XGBoost), and support vector machines are examples of nonparametric methods. “Neural nets,” more formally known as artificial neural networks, are also considered nonparametric statistics by many statisticians. Statisticians frequently utilise these methods.
Functional data analysis (FDA) is another area receiving a lot of attention. In Wikipedia’s words, FDA is “a branch of statistics that analyses data providing information about curves, surfaces or anything else varying over a continuum.” Related to this, statistical software typically offers routines for nonlinear regression. The notion that statistics is limited to straight-line associations among normally distributed variables is a misconception. There are now many approaches useful when relationships among variables are curvilinear. I make use of them in addition to methods for clustering, factor analysis and structural equation modelling appropriate for categorical and other “nonnormal” data.
Some claim that “classical statistics” cannot account for interactions (moderated effects). Actually, interactions are an important topic in any class on experimental designs or regression modelling. Nor are outliers fatal to statistics, and we now have many analytic options for data that contain extreme observations. Another odd criticism is that statistics is “backwards-looking.” What is meant by this is unclear given that “What If?” simulations, predictive analytics and forecasting have long been roles of statistics.
A further misunderstanding is that statistics cannot be used with big data. In fact, familiar statistical methods such as linear regression, principal components analysis, k-means clustering and ARIMA are very popular in data science. There is a considerable amount of work being done, however, on new methods specifically intended for gigantic data files and on faster, more efficient computational algorithms for existing methods. The latter includes Bayesian approaches, which traditionally have been very slow to estimate.
Much research is also being conducted on methods for longitudinal and time series data, which are collected over time and for which procedures designed for cross-sectional data are ill-suited. Hierarchical data where, for example, customers are nested within branches, which, in turn, are nested within regions, require special treatment as well. These kinds of data are increasingly common, and this is another frequent topic in the literature.
Spatiotemporal statistics is yet another hot area of research. Network analysis of various kinds is also an important subject in many fields. Also note that research on “old” topics such as missing data, sampling and experimental designs is ongoing.
Two areas of special interest to me are causal inference - uncovering The Why - and methods which account for multiple data-generating processes. An example of when the latter comes into play would be customer satisfaction studies (e.g., in UX, CX) in which we attempt to statistically uncover the key drivers of satisfaction. Typically, we assume one regression model (for example) is sufficient for all customers, but, in reality, there frequently are segments of customers with needs and priorities quite different from other customers. This is one reason why R-squared and other fit statistics are often disappointing - one size fits all poorly.
Regression is really a concept, not a specific technique. Anytime we attempt to explain or predict one or more outcomes (dependent variables) from one or more predictors (independent variables) with a probabilistic (nondeterministic) model, we are performing regression. Regression is essentially synonymous with supervised learning, a term popular in data science. The outcome(s) can be variables of any type - continuous, ordinal, nominal, count, censored or truncated, for instance. I only mention this because there seems to be confusion about the meaning of the word. Bill Greene’s Econometric Analysis provides an overview of regression methods popular in many disciplines.
One might assume, as I once did, that most new ideas in statistics are driven by publish-or-perish incentives and meaningless outside the Ivory Tower. This only holds a minority of papers I see, however, and I now regularly read more than a dozen reviewed journals on or related to statistics (a task I do not relish). I should also note that international interdisciplinary teams are now quite common in methodological research, and there is less “inbreeding” in statistics.
This is not the 1920s, nor is it the 1970s. A great deal of innovation has happened in the past few decades, and, if anything, the pace of change in statistics is increasing.
Kevin Gray
President at Cannon GrayCannon Gray was established in 2008 by Kevin Gray, a marketing scientist who has been in marketing research for more than 30 years. Previously Kevin had worked for The Nielsen Company's Consumer Research division, Kantar Research International and McCann Erickson as well as on the client side.
His background covers dozens of product and service categories and over 50 countries. A member of the American Marketing Association and the American Statistical Association, he is always keen to learn about innovations in marketing research and to borrow ideas from other disciplines.