Business Model Bravery
Supporting business model bravery by innovating customer research
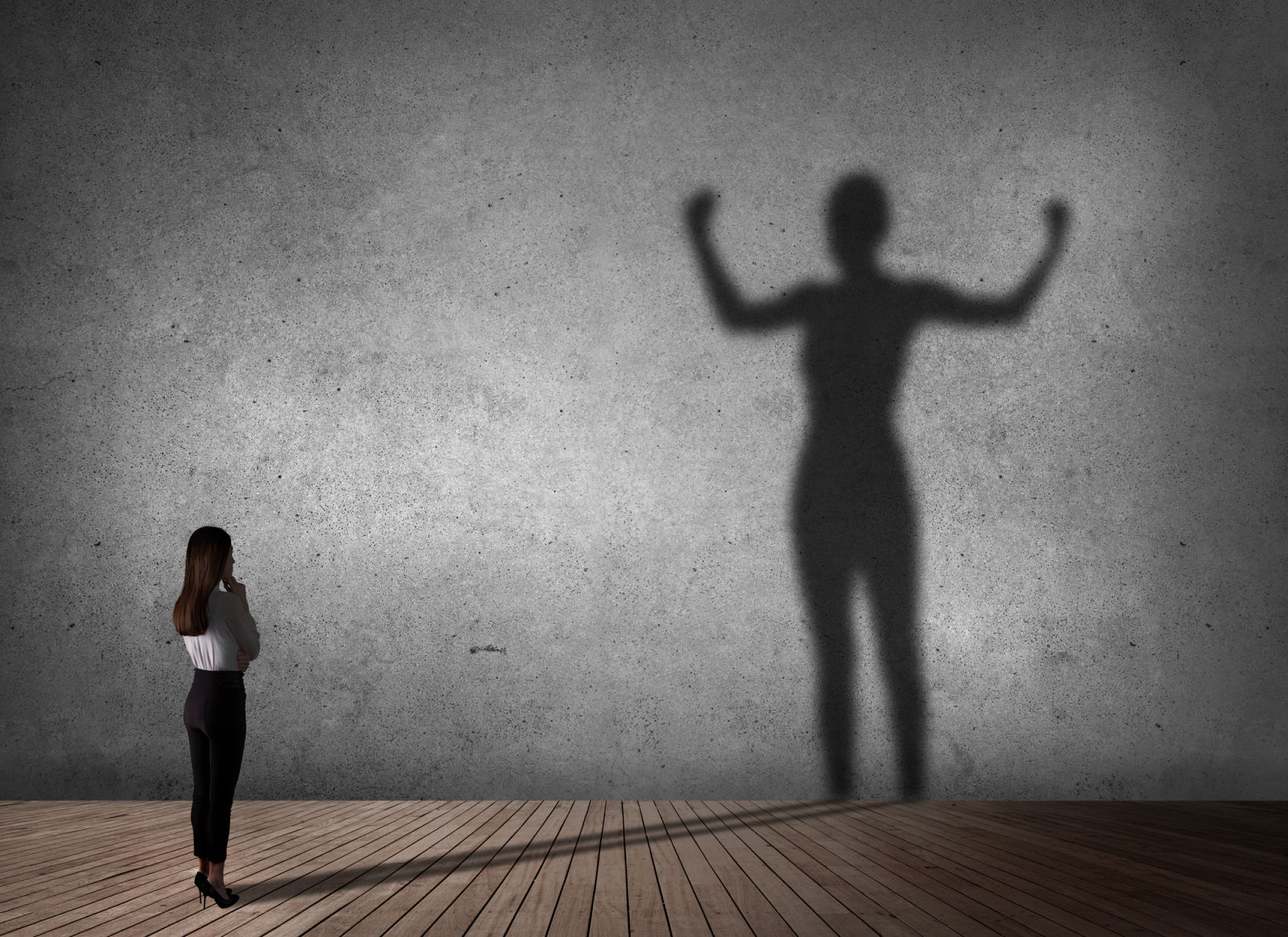
Chris Capossela, CMO of Microsoft, has talked about the importance of business model bravery in driving cloud growth (e.g. in this LinkedIn article). “One of the most important things for the way we go-to-market is to listen to how our customers want to buy and to understand how the trends are changing.” The challenge is to “find a way to move to what we think the new model is going to be, even if it could be cannibalizing our old strategy.”
In this article, we will describe how the Research + Insights team at Microsoft supports business model decision-making. We will discuss our learning journey—which we shared at this year’s Sawtooth Software Conference, for which we were awarded ‘Best Corporate Paper’—and how we strive to reflect this ‘bravery’ in our work, through innovative approaches.
What is business model bravery?
Microsoft has seen tremendous cloud growth. In 2015, we reported an annualized run rate of $5.5Bn for commercial cloud revenue. In the quarter ending in June 2022, cloud revenue was $25Bn. This growth has been assisted by a variety of business model decisions, such as:
Shifting from perpetual licenses to subscription models with Microsoft 365
Developing consumption models for ‘born in the cloud’ offerings like Azure
Embracing customer buying patterns in our business models
Monetising new categories like Windows 365 as a new cloud
The role of market research in informing business model decisions
Microsoft typically needs to make decisions on many dimensions. What payment model should we use (e.g., subscription or consumption)? With what unit (per device, per user etc.)? How should we ‘package’ the offering (add-on or stand-alone; a set of suites or individual offerings)? How should we price it? Fundamentally, how can we best meet customer needs in how they want to buy?
Customer research is a key input for these decisions. We run dozens of research studies each year, often using conjoint analysis. These business problems are typically Rubik’s Cube-like, involving thousands of possible permutations. Conjoint is a great tool here. We continue, however, to face many challenges, including:
How we assess sample quality and whether respondents can comprehend what we’re asking
How we test highly complex offerings with multiple business models and decision-makers
How we obtain realistic measures of willingness to pay, especially in new spaces
We are addressing these challenges and improving our practice through a growth mindset:
Learning: working with a broader set of research partners and industry experts, and looking at our data across studies
Innovating in our conjoint designs
Experimenting: funding work to try novel approaches, test hypotheses, etc.
Learning: sample quality
Having high quality survey samples is critical to delivering data we can trust. Historically, we have used ‘standard’ panels, but more ‘premium’ sources are also available. Is it worth the extra cost? To address this question, we did a series of experiments with ‘regular’ and ‘premium’ samples, working initially with the marketing agency Known, and then with other research partners. We found that:
The rate at which we need to reject respondents was much lower: ~50% with regular panel and under 10% with premium
Respondents were more diligent: taking much more time in the conjoint tasks
They were more consistent in their answers: giving us higher model fit statistics
The data was more credible: preference results were closer to market share, and responses to new offerings were more realistic
What is the benefit? Premium sample does indeed meet its promise. Given the cost and our volumes, we do need though to be thoughtful about usage, e.g., selecting the studies where it feels most critical, or using premium samples as a gut check on regular panel.
Innovating: conjoint designs
Next, we will illustrate how we are working to innovate our conjoint designs in ways that lead to higher quality data. An example is primary research in the PC space, in which there are hundreds of commonly purchased devices. We partnered with Known on this specific study.
We considered an adaptive approach but rejected it, in part because we were concerned about the misrepresentation of price elasticity because of selection bias. We know from exploration done by leaders in the conjoint field—e.g., in work by Tom Eagle[1]—that potential bias arises in an adaptive design if a respondent controls the presentation of alternatives. Limiting choice task alternatives to a stated consideration set alone can lead to such selection bias, which in turn may lead to inaccurate price parameters and misestimation of price elasticity.
However, we did want to present respondents with meaningful alternatives. Our solution was a probabilistic approach that borrows from the field of genetic algorithms to generate the options shown to each respondent. We asked for respondents’ consideration sets but controlled for selection bias by also presenting some devices to them from outside these sets. This approach lowered the risks of both the respondent-controlled approach and the purely random approach.
[1] Thomas Eagle, Selection bias in choice modeling using adaptive methods: a comment on ‘Precise FMCG Market Modeling Using Advanced CBC’ (2015)
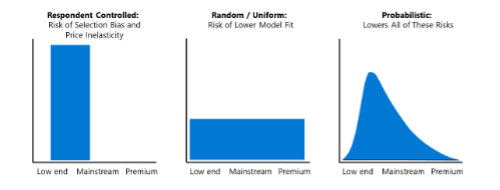
What is the benefit? In this case, a model that more accurately represented customer preferences and price elasticity of demand.
Experimenting: allocation and choice tasks, panels and online boards
With many of our commercial offerings, customers do not make single choices: e.g., they allocate workers across our Microsoft 365 plans. We decided to do two experiments, working with the market research firm Answers Research:
Experiment 1: allocation vs choice tasks. Which works better: a set of tasks involving allocations (realistic but with data quality concerns), or a set of choice tasks? We ran an experiment with cells for each approach. The choice approach produced data that was actually closer to real world share, giving us validation that the single choice approach is worth considering even in real world allocation scenarios.
Experiment 2: panel vs online boards. The second question was whether the standard panel approach worked better or recruiting a smaller number of respondents (100) and doing the exercise via online boards, a mechanism typically used for qualitative discussions. Here, the ‘standard’ panel approach worked best, but we continue to explore the online boards option. We do believe that it could be a valuable mechanism to ensure respondents do understand the tasks we present.
What is the benefit? We have a better understanding of how to approach these kinds of problems.
What’s next?
We’re conscious that this is a journey, and we remain committed to exploring ways to more accurately capture price elasticity and preference, especially in situations with complex business models. We look forward to continuing to learn, innovate, and experiment with our research partners!
Daniel Penney
Research Director (Business Planning) at MicrosoftDan leads the research team that supports Business Planning at Microsoft. This is the group that drives monetization strategy for each product. He has worked in a variety of research roles since joining Microsoft in 2004, from supporting Azure and Microsoft 365 Product Marketing to corporate issues such as leading the Customer and Partner Experience research program, and public policy research. Previously, he worked for 9 years at Research International, after taking a doctorate in history from Oxford.
Barry Jennings
Director at Microsoft, Commercial Cloud and Business Planning Research at MicrosoftBarry Jennings, Director, Commercial Cloud and Business Planning Insights for Microsoft Research and Insights group, has more than three decades of experience in market research. He started in research while still in college as a telephone survey researcher and later worked on international technology brand-related market research at Millward Brown IntelliQuest. He spent 18 years at Dell in research positions that covered almost every product the company produced. Next, Barry moved into the mobile space by joining BlackBerry, where he was head of market research and competitive intelligence focused on business to business solutions. At Microsoft, Barry and his team deliver insights to drive the company’s cloud marketing and commercial business planning groups. Barry’s research focus includes cloud computing, data platform, AI, development tools, and other enterprise solutions.