Synthetic data: A game-changer in data-driven decision making
Synthetic data is starting to gather momentum in the insight industry. Creating and using synthetic data isn’t just a response to the increasing scarcity of real-world data. It is, in fact, a strategic tool designed to bridge significant data gaps.
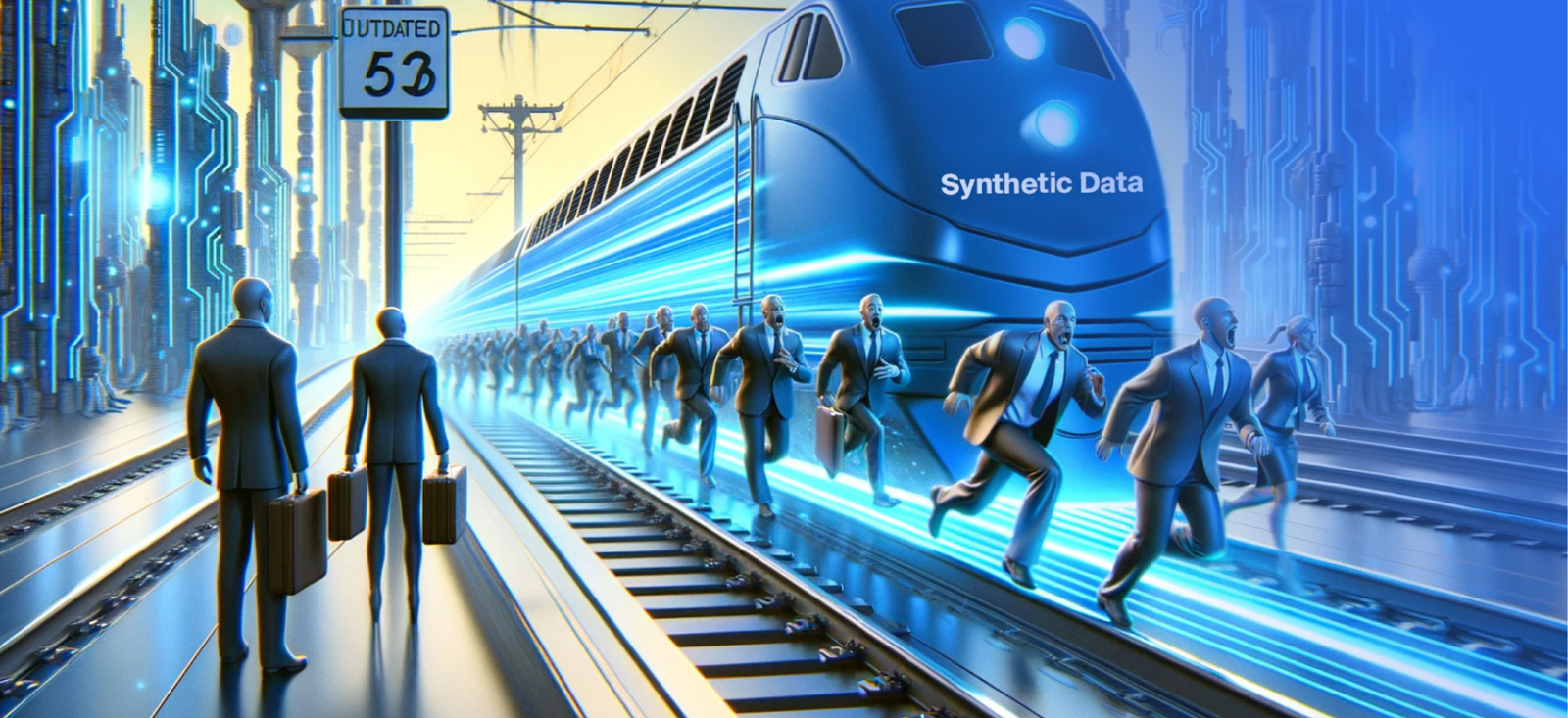
Synthetic data is starting to gather momentum in the insight industry. In a recent article published in Research World, synthetic data was explored with a hint of skepticism, particularly regarding its potential misuse and the perils it could pose if it were to supplant original data collection methods.
This critique demonstrates age-old reservations about technological advancement. Humans are naturally averse to change. Especially when what’s being changed is an established practice – in this case data collection. This article aims to delve into these reservations and help researchers see the potential opportunities synthetic data offers.
Synthetic Data as a Strategic Tool
Creating and using synthetic data isn’t just a response to the increasing scarcity of real-world data. It is, in fact, a strategic tool designed to bridge significant data gaps. For example, dynamic AI-driven personas can emulate real customer interactions with accuracy, delivering insights that would traditionally require extensive fieldwork.
Instead of depending exclusively on surveys or human participants, generative AI acts as a substitute for human input by utilizing vast datasets – normally more broad, up-to-date and accurate than those obtained through traditional research gathering mechanics.
This method facilitates the exploration of new ways to acquire insights and expands the range of possible questions. Consequently, Virtual Audiences provide immediate, comprehensive insights across various topics, markets, and demographics by dynamically processing data from multiple sources.
It’s important to know that this isn’t ‘fake data’ – it is AI-generated data that is synthesized and derived from very real and reliable data across a plethora of trusted sources, including traditional survey data, publicly available statistics, trend reports and more. In a rapidly evolving market landscape, such real-time data synthesis is not just beneficial; it’s becoming increasingly necessary.
The Evolution of Data Richness
Detractors argue that synthetic data might disconnect us from reality, but they’re overlooking the fact that Generative AI doesn't just amass data; it breathes life into it. This allows it to grow and adapt over time, ensuring continuous relevance and accuracy. And its accuracy is not only comparable to traditional data, but competitive with it. In a recent article in Marketing Week, marketing aficionado Mark Ritson finds that "most of the AI-derived consumer data, when triangulated, is coming in around 90% similar to data generated from primary human sources."
Augmented Data can further insight generation by integrating diverse data sources, including proprietary datasets, academic content, and real-time web searches, with advanced machine learning algorithms. This method transcends traditional surveys and human feedback, utilizing generative AI to synthesize data into comprehensive knowledge lakes. In this model, data amalgamation serves to create detailed personas, formulate relevant questions and answers, and distill key insights, offering users a nuanced, multi-dimensional view of their topics of interest and delivering precise, AI-driven insights.
Addressing the Challenges with Traditional Sample
It’s no secret that the market research industry has a data quality challenge with traditional sample panels. Data quality is by far the most important factor when choosing a market research partner or supplier (GRIT Report 2020). Traditional panels are universally applying belts and braces to try and address these challenges, including working with researchers to support better survey design, however, we believe users of research should also be looking to alternative data sources such as synthetic data.
Synthetic data can help address the issue of sample quality that often plagues traditional survey data in market research. Traditional sample data frequently contains superficial responses, erroneous entries or lazy respondents, even after all of these belts and braces have been applied. This has the potential to significantly skew the findings and reduce the fidelity of the dataset.
Conversely, synthetic data, sourced from real datasets and engineered through sophisticated algorithms, presents a cleaner, more controlled set of insights. It minimizes the noise and irrelevant information often found in survey responses, ensuring that the data harnessed is of high quality. Market researchers can then delve into a realm of precision, unencumbered by the typical inaccuracies or superficialities associated with traditional sample data.
This artificial generation of data also helps in mitigating unconscious biases that human data collectors might introduce, as it relies on predetermined rules and parameters rather than subjective judgment. There are of course biases inherent in LLMs, but these are generally identifiable and can be adjusted for, they are not subconsciously contributed by survey respondents or survey design. Moreover, synthetic data can fill gaps in existing datasets, providing a more holistic and inclusive view of populations.
Research Innovation and Efficiency
Synthetic data is already starting to make research cheaper and more scalable. Traditional data collection methods often involve lengthy processes of gathering, cleaning, and validating data, which can be both time-consuming and costly. Synthetic data, on the other hand, can be generated quickly en masse and, tailored to specific research needs. This means that researchers can access a vast array of data in a fraction of the time it would take to collect real-world data.
Being able to create data that mimics real-world scenarios without the need for extensive fieldwork or surveys allows researchers to focus on analysis and interpretation rather than data collection.
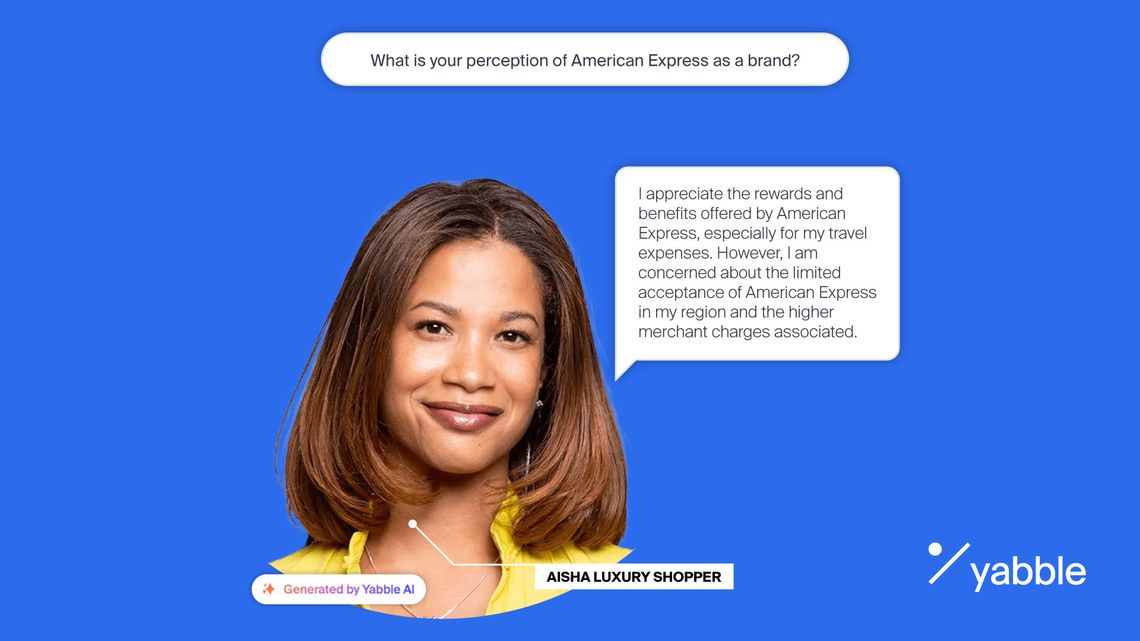
Moreover, synthetic data offers significant advantages in terms of data quality and consistency, which are crucial for operational efficiency in research. In traditional data collection, inconsistencies and gaps are common, often necessitating additional rounds of data collection or complex data cleaning procedures. Synthetic data can be programmed to adhere to specific quality standards and to be free from common data issues such as missing values or outliers, ensuring a higher level of congruency. This congruency means that researchers can trust the data they are working with, reducing the time spent on data verification and preprocessing. The ability to simulate various scenarios and conditions also allows for more comprehensive testing and validation of models and hypotheses, leading to more robust and reliable research outcomes.
The Path Forward with Synthetic Data
While skepticism towards synthetic data is a natural response to a transformative technology, it’s crucial to recognize and embrace these innovations, especially when considering their accuracy, relevance, and utility.
As we continue to navigate the discourse surrounding synthetic data, it’s evident that technology is evolving how we approach data. Its potential to redefine market research and a myriad of other domains is vast. With responsible utilization and continuous innovation, synthetic data stands poised to become an integral component of insightful decision-making in our digital era, complementing and in some cases, enhancing traditional data collection methodologies.