Artificial Intelligence for Consumer Insights
This article acknowledges the leaps AI has made for our industry but also highlights the pitfalls of distancing ourselves from the people we seek to understand.
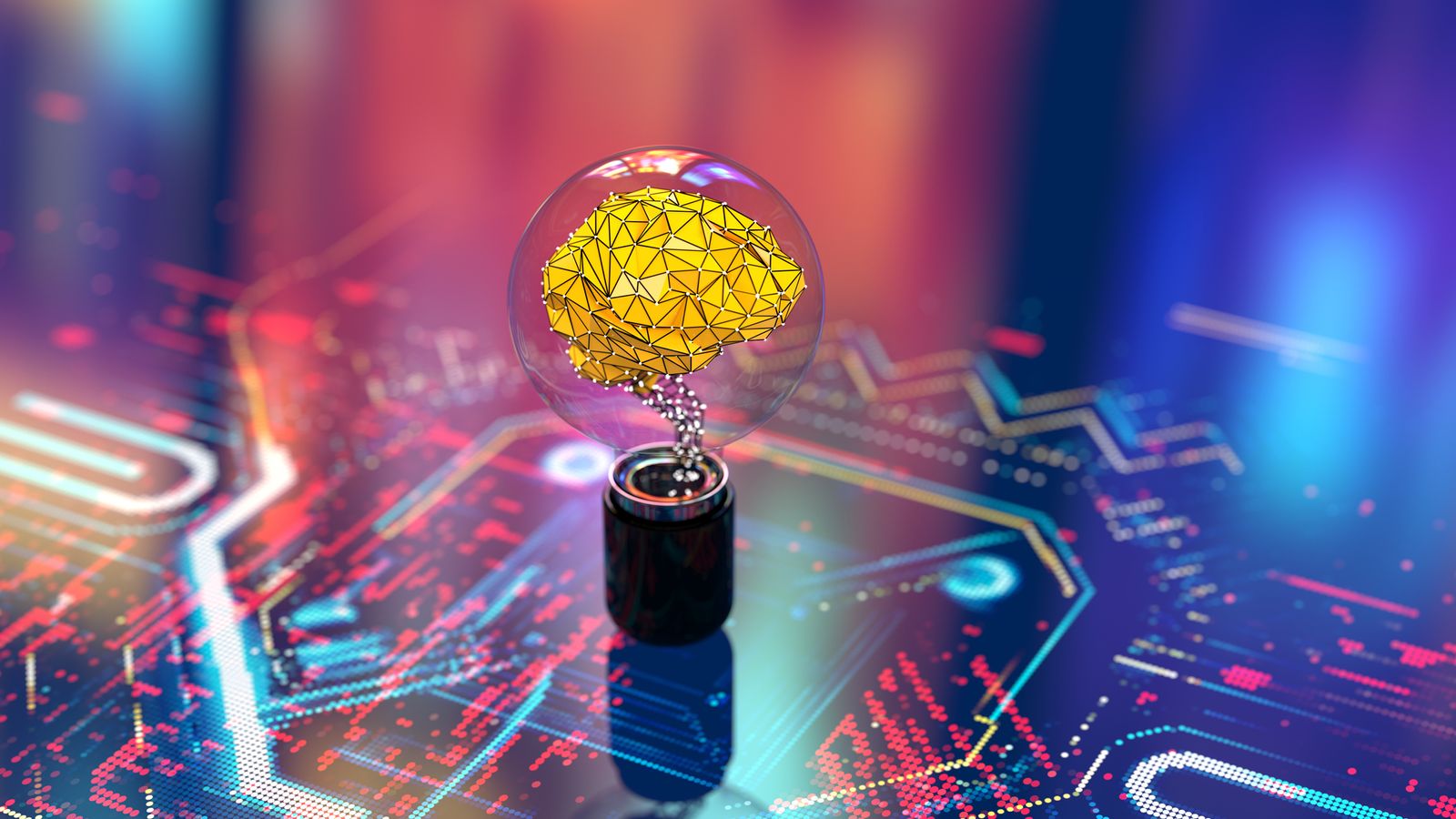
Drop into a market research conference or open an industry newsletter, and you are likely to be inundated with buzzwords around the potential of artificial intelligence. Many research agencies (ourselves included) have incorporated AI tools as part of our repertoire. This article acknowledges the leaps AI has made for our industry but also highlights the pitfalls of distancing ourselves from the people we seek to understand.
How can AI help the insights industry?
Brands primarily use AI tools in 3 categories: process automation, cognitive insights, and cognitive engagement. The second bucket – cognitive insights – which helps give meaning to large amounts of data, is most relevant for the customer insights industry.
In particular, predictive analytics is an area where machine learning has shone through for decades. An early example is Target knowing when customers were pregnant before they even knew this themselves, based on metadata around specific purchase changes in the early stages of pregnancy. Later models are still used today, for example, when Netflix recommends what you should watch next, or Amazon recommends products for you to buy.
A second key application in our industry is analysing social media data to build consumer sentiment data using Natural Language Processing (NLP) algorithms. Using data feeds generated by NLP algorithms to help build in culture analysis around specific demographics, geographies, and consumer behaviour.
A third application is to outsource the grunt work – think cleaning data sets or generating charts for quantitative consumer insights data.
Is AI going to take over our jobs?
In a nutshell, no. The key problem with AI algorithms – widely acknowledged in multiple spheres – is human bias corrupting datasets that AI tools are built on. Or, as they say in the computing world, ‘Garbage In, Garbage Out’.
Fundamentally, AI algorithms are only as good as the data that is fed into it. This has led to numerous problems stemming from human biases creeping into dataset creation. Famous examples include racial bias in facial recognition algorithms leading to arrests of innocent people of colour and Google Photos mis-labelling people of colour as gorillas.
In the consumer insights world, relying on AI creates a big blind spot: building on data that is familiar to researchers like us (predominantly people with privilege), thus ignoring consumers and cultures that are relevant for brand expansion.
Where AI falls short: Helping brands break into new markets
As a behaviour and culture insights agency, much of our recent work has focused on established brands expanding into new segments and markets. Typical brand strategy questions involve understanding how to market to the bottom of the pyramid consumers, expand to new countries, or break into unfamiliar subcultures like gaming.
Our work in this space has rested on the premise of breaking out of preconceived notions and recognising our personal biases as urban, privileged researchers. Participatory research approaches are critical to understanding new spaces, and transferring power to the hands of communities we seek to understand instead of being top-down researchers. For example, having community members lead the research, creating videos about their lives, or being guided through immersive tours of their contexts.
These are nuances lost in AI research tools – for instance, even social media data analysis is limited by algorithms' understanding of local language scripts and different phrases of expression.
As we move towards a future where human researchers co-exist with AI tools, we need to stay true to our human core as researchers. We need to step outside our comfort zones and experience unfamiliar ways of life – by visiting new areas, conversing with different types of consumers, and understanding their perspectives and beliefs. This will help ensure that we are not distanced from ‘real life’, both from an AI perspective and through a reflection of our own human biases.
Sanaya Sinha
Lead Insight & Design Strategy at Quantum Consumer SolutionsSanaya is an experienced qualitative and quantitative researcher with data science skills. At Quantum, her work has spanned a range of categories including FMCG, nutrition, personal care, and technology.
She has also worked in the development and policy research sectors, with a focus on sanitation, adolescent health, nutrition, and public finance.