The evolution of research methods
Over two round table sessions, we (a collection of clients and suppliers from a wide range of countries, including Japan and Tunisia) discussed the implications of AI on the evolution of research methods.
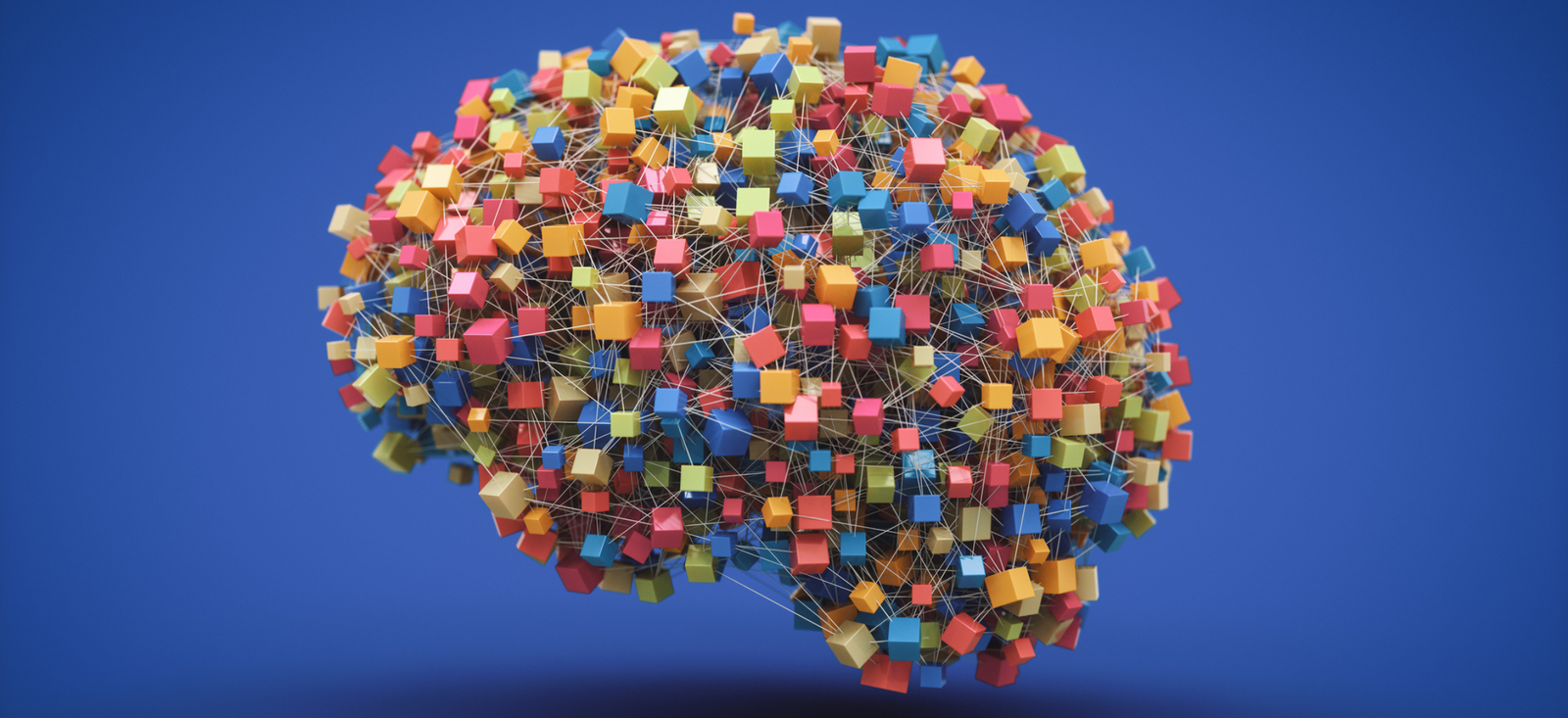
Article series
AI Taskforce Roundtables
- The evolution of research methods
- Enriching qualitative insights
- Unleashing AI's power in quantitative analysis
- Crafting compelling narratives from AI-generated Insights
- Adventure Table - GPT Exercise: Practical AI Engagement with ChatGPT
- Pros & cons: The dual faces of AI
- Bias and ethics of Artificial Intelligence
Over two round table sessions, we (a collection of clients and suppliers from a wide range of countries, including Japan and Tunisia) discussed the implications of AI on the evolution of research methods.
What might not change?
As part of assessing the evolution of research methods, we discussed things that are less likely to change in the near future. The key element that was felt to remain constant was anything where the human touch was at a premium. Examples included human interviews where empathy and depth were needed, working with clients to understand research and business questions, and utilising storytelling to communicate insights.
In terms of analysing qual, there could be a dichotomy between AI looking at large amounts of qualitative data (albeit shallowly) and deep human analysis, working with smaller amounts of qualitative data, probing for deeper truths. Things like human-based ethnography will still be needed.
If Generative AI stays very American, most cultural analysis will still need to be human-centric.
Research design
Some of the group reported using Generative AI to aid their brainstorming, concept designing, and questionnaire creation. Another aspect of research design will be using AI to test and evaluate questionnaires and discussion guides.
Smart data collection
Many clients use self-serve/DIY systems, which still require the user to design the right project, write a good questionnaire, select a suitable sample and conduct the analysis. AI will create smart DIY systems, co-creating the research, fielding the study, and conducting the basic analysis. This will make self-serve/DIY much safer and allow a wider range of people to conduct research without specialists and agencies.
More qual
AI allows more open-ended data, images, and videos to be processed. This suggests more qual data will be collected. This change creates a shift from focusing on what can be easily measured to what is likely to generate insights. This increase in the amount of qual being conducted is already apparent in the 2023 ESOMAR Global Market Research Report.
Synthetic data
The focus of synthetic data is faster and cheaper, which can widen the range of situations in which research is used. One of the questions we discussed related to how long synthetic data may stay ‘in date’. For something like virtual eye-tracking (which is based on typical human behaviour) the synthetic process might last years, but views about specific brands in the market might have a much shorter shelf life.
In markets where CAPI and CATI are still major factors, such as Tunisia, we can see synthetic data making a massive speed and cost difference – synthetic does not need to be only constructed from online data collection. Synthetic data approaches can be used to reduce the size of current questionnaires, collecting datasets with large amounts of missing data, which can be estimated.
Secondary data
AI can be the key to unlocking the data clients already have. The first level is using AI to combine and organise disparate data sets. Next, AI will allow the user to use natural language queries to search databases for information. If complete answers are not available, the system can then interpolate information.
New solutions?
In both sessions, we asked the members from the client side about the sorts of problems that current MR struggles to answer. The main answer was competitor analysis. What do their competitors' customers think, and how do they see the world? Could synthetic data be a partial answer to this need?
We see that Hollywood is using Generative AI to write screenplays and create virtual actors and voiceovers. Could this be used to create better debriefs, presentations, and ‘leave behinds’? Using AI to create predictive and prescriptive models would also be a great extension.
Changes to the Insights ecosystem
Smart DIY will increase the amount of insights conducted internally by clients. The increase in the use of internal insights is likely to be via managers, designers, etc, rather than by insight teams. It is unclear whether the key winners on the supplier side will be a) the large agencies, who can create large, global, AI-powered solutions, or b) small agencies focusing on consulting and leveraging AI to do the heavy lifting.
A future client scenario?
In a client meeting, somebody asks a question to the AI related to insights.
Step 1) Do we already know the answer?
Step 2) If we don't know the answer, can we interpolate it from the information we have?
Step 3) Can we use synthetic data to estimate an answer?
In all these scenarios, the team gets the answer in minutes. The AI then asks the team if they want to ‘validate’ the answer. If the team say yes to the validation, the AI writes a survey, fields it, analyses it, updates the database, and sends an update to the team; in this scenario, the validation might arrive, say, 48 hours afterwards.
Ray Poynter
President at ESOMARRay has spent the last 45 years at the intersection of insights, research, and new thinking. Ray has held director-level positions with companies such as The Research Business, IntelliQuest, Millward Brown, and Vision Critical. Ray is committed to the research and insights industry, having been a member of ESOMAR for over 30 years and a fellow of the MRS.
In recent years Ray’s work has focused on training, writing, speaking and sharing. Ray has run training workshops for a variety of national and international organisations, including RANZ, TRS, JMRA, MRS and ESOMAR. Ray has written textbooks, taught at Saitama and Nottingham Universities, regularly blogs, and is active on social media.
In 2023, Ray was elected President of ESOMAR.
Article series
AI Taskforce Roundtables
- The evolution of research methods
- Enriching qualitative insights
- Unleashing AI's power in quantitative analysis
- Crafting compelling narratives from AI-generated Insights
- Adventure Table - GPT Exercise: Practical AI Engagement with ChatGPT
- Pros & cons: The dual faces of AI
- Bias and ethics of Artificial Intelligence