The growing role of data science
The ever-expanding role of data science in driving decisions and shaping strategies within organisations
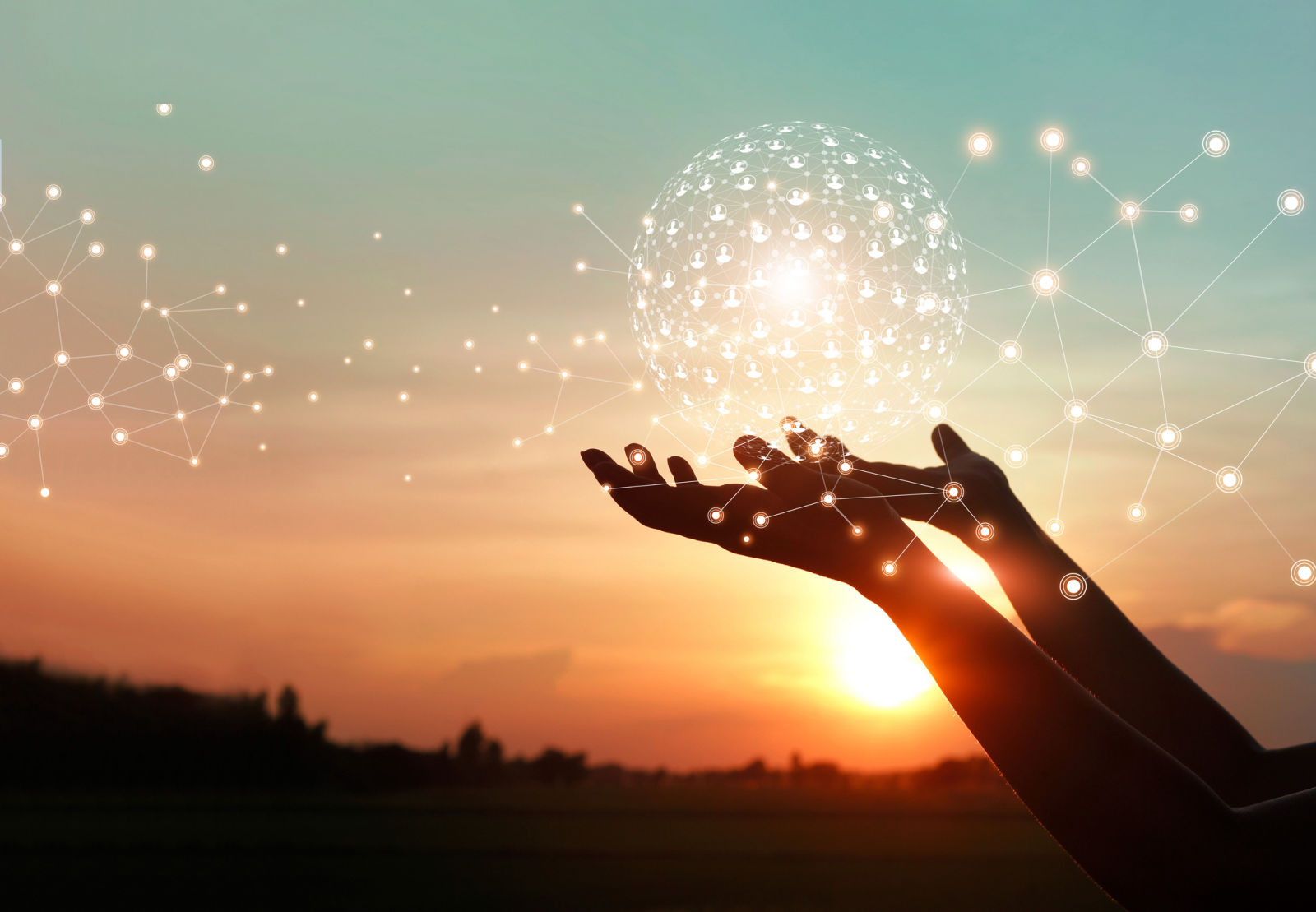
The Insight250 spotlights and celebrates 250 of the world’s premier leaders and innovators in market research, consumer insights, and data-driven marketing. The inaugural list was revealed last April and created renewed excitement across the industry whilst strengthening the connectivity of the market research community.
With so many exceptional professionals named to the Insight250 it seems fitting to tap into their expertise and unique perspectives across an array of topics. This weekly series does just that; inquiring about the expert perspectives of many of these individuals in a series of short topical features.
This edition reviews the ever-expanding role of data science in driving decisions and shaping strategies within organisations. I sat down with Kyle Findlay to get his expert perspective on the topic. Kyle serves as Senior Data Science Director for Kantar in South Africa. We discuss how data science is influencing a spectrum of groups across the average company and is seeing its importance grow as markets and consumers change more rapidly.
Lots of people talk about “data science,” which can often mean different things, how would you describe data science?
“For me, ‘data science,’ as a field, uses statistics and programming to extract patterns and understanding from data. It does this at scale and often to support a ‘data product’ which leverages the methodologies designed and implemented by data scientists to provide end-users (people and machines) with insights or direction.”
Kyle Findlay
There’s increasing talk of using data science alongside insights but what does this really mean and how do these disciplines add value beyond primary research?
“Data science adds value to so many parts of the insights value chain. It allows us to extract insights from new data sources (such as unstructured text, images, video, and more); it allows us to extract patterns from larger datasets; it allows us to automate many statistical processes and build on top of them (or daisy-chain them together) in order to make more complex and valuable data products; it allows us to make better forecasts that are informed by more data and that use more advanced techniques (such as neural networks); it allows us to do data fusion across datasets to give us a more holistic picture of the market and consumers; it allows us to have more naturalistic interactions with consumers through chatbots and other modalities; it allows us to engage consumers through greater personalisation and real-time responding; and more.”
Kyle Findlay
What is driving the evolution of "data science" - is it technology, methodology, data integration, analysis, etc.?
“Without a doubt technology and methodology, although the other areas play a role too. Research institutions (both academic and commercial) release new methodologies and models of the world for data science teams to leverage on what seems like a daily basis. Just this past week, Facebook released a model (GPT-J) into the public domain that has an amazing understanding of language and can be used for many tasks. These models are built on novel tweaks to existing methodologies and those organisations have unprecedented access to data, and they often share these with the data science/AI community.
“This space moves fast and is ever-changing. At the same time, it is becoming ever easier to create AI models, methodologies, and products thanks to technology platforms like Microsoft Azure, Amazon AWS, Google Cloud Platform, and a host of other startups. They take a lot of the grunt work out of data science tasks, the deployment of models, and the access of them by other people and products; all of which are working to democratise these abilities. At the same time, your data science product is only as good as the data you have access to, so data integration is important here, and more and more organisations are realising this, making data more governed, mastered and accessible. Finally, even analysis is taking leaps forward due to progress in areas like NLU (Natural Language understanding) and NLG (Natural Language Generation) which allow machines to understand data, intent, etc. and generate text that adds meaningfully to analysis. Similarly, data exploration and visualisation tools also make analysis easier and better. It’s a fast-evolving field that is disintermediating many gatekeepers.”
Kyle Findlay
Are advances in data science allowing our profession to deliver results “better, faster, and cheaper”?
“Sometimes yes, sometimes no. I have yet to see a data science output that can automatically produce outputs to the standard of a research professional. Rather, such tools augment research professionals, allowing them to get somewhere faster or do more with the same resources. What is also happening though is that people are becoming familiar with the limits of ‘AI’ and sometimes moderate their expectations of it, creating a tiered system where some clients are happy with ‘good enough’ AI outputs, while others insist on “research-grade” outputs that require a human-in-the-loop scenario. There’s space for it all.”
Kyle Findlay
When recruiting data scientists, how do you balance the need for specialists and those with broader business awareness? Is commercial acumen as important as an understanding of data science?
“This is a perennial debate. Very few data scientists have deep domain knowledge outside of their technical area. This is not new though. The same exists in most software development teams, for example. Generally, this is managed through an Agile workflow where different stakeholders each have a role to play in creating something that is technically strong but also relevant to the organisation’s business needs.
“A good Agile process should have strong guiding input from Business Analysts who act as the organisation’s business representative and understand its needs, and, with the help of Project Managers, ensure that these needs shape the technical outputs that are produced in a virtuous feedback loop. It’s when one starts to compromise on the Agile process that things can start to veer off course. Therefore, a data scientist doesn’t need to know the business (although it definitely helps a lot!) as long as you have a strong team in place that follows good processes.”
Kyle Findlay
Generating great, evidence-based, insights from the data is fantastic, but, how do your team “tell the story” to get the findings actioned within the business?
“The first thing to do is to ensure that what you build speaks to an existing business need. Staying on track with this need will help you get immediate buy-in since business stakeholders will have been involved from the beginning. In addition, there’s always a place for good storytellers and synthesisers of data. Someone who can grok the data and identify the spine of the story is a unique and hard-to-find skill to find. Then, an important part of building any data product that is sometimes ignored is the logging and monitoring of that product. Who is using it? How often? Which parts? Etc. This allows you to close the loop and demonstrate the impact of what you build and the role it’s playing within the organisation, or to pivot to something more relevant.”
Kyle Findlay
What do you see as the biggest innovations coming in data science and how will they help our profession?
“The massive models that capture language and other modalities continue to blow my mind. I feel like I am living in the future when I see models like DALL-E which generates images (and soon videos I am sure) from text, and giant language models like GPT-3 and GPT-J which allow us to converse with machines, allow machines to understand us, etc.”
Kyle Findlay
As a respected leader in data science, where do you learn from/who do you look to for thought leadership and to champion your discipline?
“I wouldn’t call myself a data scientist to be honest. Rather, I am a data product builder and I lean heavily on the different disciplines within my team from data scientists, to data engineers, developers, etc. I’m always interested in what the ‘godfathers of AI’ like Yann le Cun, Yoshua Bengio, and Geoff Hinton are saying though. Also, organisations like Hugging Face, OpenAI, DeepMind, etc. The usual bunch I guess for anyone in this area. Unfortunately, it’s often the very large organisations only that have access to the resources to do the really cutting edge (or is it just really large-scale?) stuff. Luckily, the likes of Microsoft, Facebook, Google, etc. tend to share a lot (or rather some) of what they are doing with the public.”
Kyle Findlay
Hot Topic: The next generation of leaders
Kyle - you’re a guest lecturer at the University of Cape Town - how do you believe the next generation of leaders view our profession? How can we better engage with them to highlight the fascinating careers that exist within our profession?
“I wouldn’t say that I have my finger completely on the pulse in this area as my lecturing has been more in the media domain. Having said that, what I would impart on new insights professionals is the amazing data, models and frameworks that we have available to help us understand why people do what they do. There are not that many fields that allow you to be a psychologist, statistician AND data-driven story-teller. I’d impart upon them the emergence of these new data modalities beyond survey (which really still has its place) to help us make sense of the world for ourselves and our clients. It’s the insight into human behaviour that has always kept me engaged with the insights industry.”
Kyle Findlay
Top Tip
“Don’t assume that everyone knows better than you or that all problems are solved; there’s often a better way of doing it and, if you build the right networks, and surround yourself with the right people, you can do cool things and make money.”
Kyle Findlay
Very interesting perspectives Kyle – thank you for sharing. Clearly, as the speed and complexity of evolution in the data space increases, understanding the changes impacting markets and products in real-time and how consumers react to these changes is largely the responsibility of data science. Individual experts, like Kyle, are leading their teams to develop solutions that can manage the volume, variety, and velocity of data in order to discover insights that can drive decisions and shape strategies. With machine learning and artificial intelligence expanding their capabilities the role of data science is becoming increasing valued across organisations.
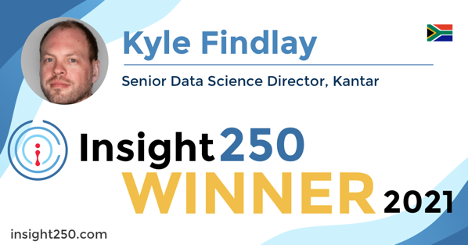
Crispin Beale
Chairman at QuMind, CEO at Insight250, Senior Strategic Advisor at mTab, CEO at IDXCrispin Beale is a marketing, data and customer experience expert. Crispin spent over a decade on the Executive Management Board of Chime Communications as Group CEO of leading brands such as Opinion Leader, Brand Democracy, Facts International and Watermelon. Prior to this Crispin held senior marketing and insight roles at BT, Royal Mail Group and Dixons. Crispin originally qualified as a chartered accountant and moved into management consultancy with Coopers & Lybrand (PwC). Crispin has been a Board Director (and Chairman) of the MRS for nearly 20 years and UK ESOMAR Representative for c15 years. As well as being CEO of Insight250, Crispin is currently Worldwide CEO of Digital Communications Solution Agency, IDX. Crispin is also the Senior Strategic Advisor at mTab and the Chairman of QuMind and spent 4 years as Group President of Behaviorally where he was responsibile for the client & commercial teams globally. Crispin is a passionate advocate for blending human intelligence and technology to deliver innovation and leadership across organisations.