Synthetic Data - Just a hype? Decoding Synthetic Data for Product Testing
This Ipsos study on synthetic data in product testing emphasises its potential to enhance human data collection when traditional methods are costly.
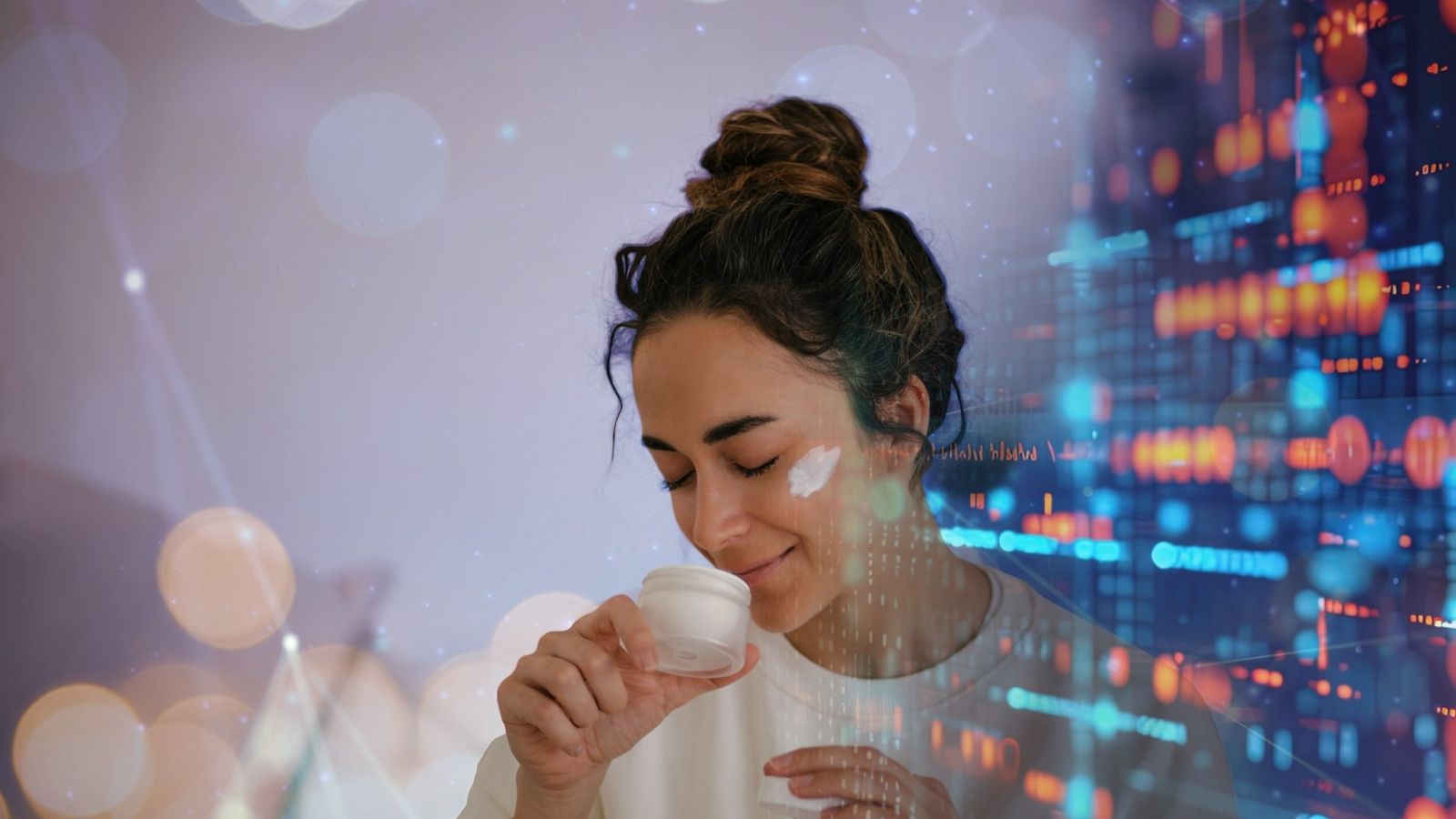
This article explores the use of synthetic data in product testing, drawing insights from a study conducted by Ipsos. It examines the potential of synthetic data to augment human data, particularly in scenarios where traditional data collection is costly and time-consuming. The study investigates the conditions under which synthetic data can accurately replicate results obtained from real-world data, focusing on the trade-offs between cost, time, and accuracy. The findings suggest that while synthetic data offers significant advantages, particularly in augmenting smaller human samples, its effectiveness is contingent on the quality of the training data and the nature of the products being tested.
Synthetic data, artificial data generated from models trained on real-world data, is rapidly gaining traction across various industries. Its potential to accelerate drug development, simulate financial transactions, and test autonomous vehicles has been widely recognized. In market research, synthetic data offers new possibilities, particularly in product testing. However, many businesses remain uncertain about its quality and evaluation. This article addresses these concerns by presenting Ipsos' insights into testing products with synthetic data, focusing on data augmentation, which involves enhancing respondent-level datasets with synthetic data.
Generating and Evaluating Synthetic Data
The generation of high-quality synthetic data requires careful consideration of two key aspects: the training process and the evaluation of the generated data. An AI must be trained on real-world data relevant to the business to generate synthetic data that accurately reflects real-world statistical properties. The evaluation process involves comparing synthetic data with real-world data on common statistical measures such as means, distributions, variances, and correlations. The closer the synthetic data is to the real data, the lower the risk associated with its use. However, it is crucial to acknowledge that synthetic data can never perfectly mimic real data in every aspect, and its use should be considered when some risk is acceptable.
Approaches to Generating Synthetic Data
Two primary approaches exist for generating synthetic data: Large Language Models (LLMs) and non-LLM methods. LLMs, pre-trained on extensive datasets, can generate high-quality synthetic data in areas covered by their training. However, they have limitations, including limited coverage, biases towards Western, English-speaking countries, and outdated information. Therefore, training LLMs on updated, country-specific real-world data is crucial for generating high-quality synthetic data. Non-LLM methods, particularly Deep Learning (DL) algorithms, excel at generating numeric synthetic data that closely mirrors the statistical properties of real data. Unlike LLMs, DL algorithms do not have pre-trained models, allowing for a blank slate approach where the algorithm is trained on current, market-specific human data. In addition, DL allowed us to create new respondents without just replicating existing respondents which would violate rules of statistical testing, such as in Boot Strapping. Other means of synthetic such as weighting does not increase the sample size.
The Rationale for Product Testing with Synthetic Data
While synthetic data offers anonymity advantages in other fields, the primary benefit for market research is cost and time savings. Product testing is particularly suitable for synthetic data due to the high costs associated with manufacturing, shipping, and sampling. However, the trade-off between cost savings and accuracy must be carefully considered. In situations where the cost of conducting research is high, synthetic data can be a valuable tool.
The Role of Human Input
Despite the potential of synthetic data, human input remains essential. AI alone cannot capture the nuances of human sensory experiences, emotions, and expectations. The goal of applying synthetic data to product testing is not to replace human input entirely, but to augment it. The challenge lies in determining the minimum number of human respondents needed to test products alongside synthetic data while ensuring viable results.
Research Streams and Findings
Ipsos conducted two research streams to investigate the use of synthetic data in product testing. The first stream analyzed more than 80.000 consumer responses across categories and countries and determined that a sample of 50 human respondents is sufficient to replicate the performance rankings of the best and worst products when the difference is at least 8%. The second stream validated that small human samples, when augmented with synthetic data, yield similar results to all-human samples. The study covering 6 different countries and 9 different categories found that the two datasets (pure human data set of n=200 per product vs augmented dataset with n=50 real human +150 synthetic) were remarkably similar in terms of product performance rankings, data distribution, and relationships between variables. Most importantly, the two datasets led to the same business decision, despite some differences in variances.
The Benefits of Augmentation
A key benefit of product testing with synthetic data is the ability to augment data for hard-to-reach populations. This can lead to statistically significant differences that were not apparent in smaller all-human samples. For example, augmenting a sample of brand users with synthetic brand users can reveal statistically significant differences between products that were not previously detectable.
Cautions and Considerations
The ability of a part-human, part-synthetic dataset to replicate the findings of an all-human dataset depends on several factors:
Representativeness: The human seed sample must accurately represent the target population. Misalignment can lead to synthetic data that fails to capture true product performance.
Product Differentiation: Significant differences between products enhance the AI's ability to replicate these distinctions in synthetic data.