Synthetic Data – Get on Board but do it wisely!
(A written debate from Crispin Beale, Simon Chadwick, Mike Stevens and Finn Raben)
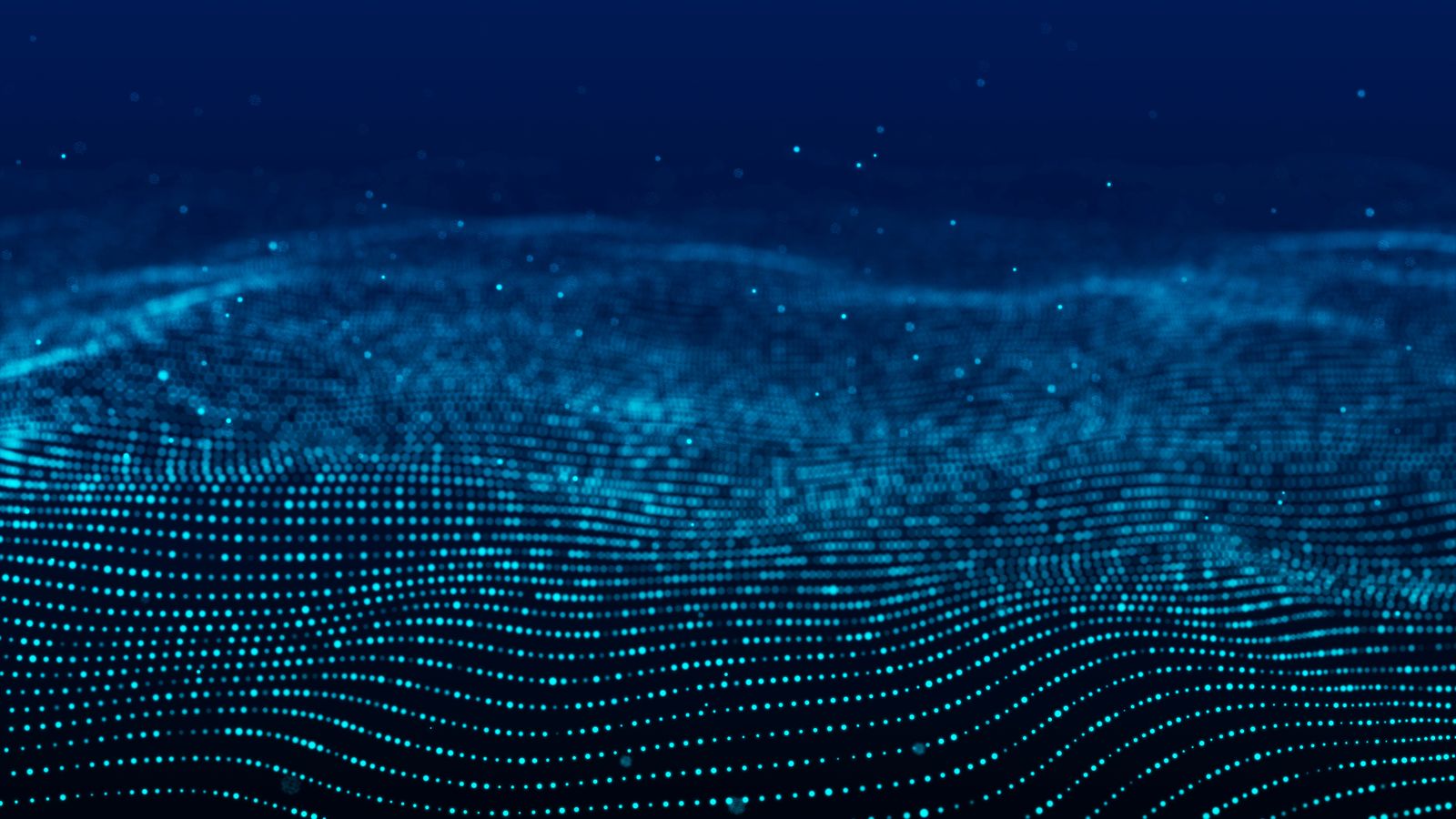
Firstly, an observation: the profession of research has always been founded on three primary principles – i) rigour (triangulation/validation/contextualisation), ii) objectivity and iii) transparency. Yet, many of our profession’s “new” approaches are all too often assimilated into our toolbox without being subjected to these stress tests, thus leading to significant, unintended consequences.
As an example, many of us forewarned more than a decade ago (probably two!) about the risks of declining participation rates and respondent quality. To alleviate these concerns, we embraced panels and inadvertently started a “race to the bottom” in terms of cost, and importantly, quality. We are still struggling with these issues. Our industry has a “history” of embracing or diving head-first into new concepts, taking the benefits, but not always assessing the longer-term impacts.
Synthetic data presents us with a similar opportunity AND challenge - Let us NOT repeat our mistakes of the past?
A historical analogy
Less than 100 years ago we heard about polyester, a game-changing new synthetic fibre that would revolutionise clothing. It was advertised in the 1960’s as “a miracle fibre that could be worn straight for 68 days without ironing and still look presentable”.
Polyethylene terephthalate (PET), generically known as polyester, entered commercial production in the United States in the early 1950s, since which time – due to its durability, resistance to shrinking and stretching, and ease of care, it has now become one of the world’s most popular textiles, used in thousands of different consumer and industrial applications.
Today though, we know that the “miracle” had unintended and unforeseen consequences.
It is estimated there are 14 million tonnes[1] of microplastics on our ocean floors and that this number is growing by 500 thousand tonnes each year. Roughly, one-third of this pollution comes from synthetic clothing when it is washed, as microfibers escape, polluting our planet and bringing environmental consequences we are only now starting to understand.
The human race has been slow to respond, in any meaningful way, and only now are companies such as Patagonia, Samsung Electronics and Ocean Wise collaborating to find ways of reducing this ongoing build up (eg Samsung’s filters for washing machines that remove just over half (54%) of these pollutants before they reach the oceans, but effective, global solutions to deal with the problem are still non-existent.
Synthetic data (or synthetic respondents)
Like “Big Data” and other trends before it, synthetic data has now captured the profession’s imagination and is being explored and potentially embraced across our sector in a variety of ways. While synthetic data is not a monolithic or isolated innovation (it is, after all, a component part of a multitude of different innovations), it is most commonly presented as a binary choice in opposition to “real” or “survey” data, which we all know carries its own challenges. That said, for this article, we will debate synthetic data on the premise of that binary choice.
Firstly, synthetic data is not “new”, we’ve been using it for years and so should be well aware of its limitations. However, our passion for investing in and adopting new technologies or “toys” should not stop us from applying our three foundational principles, lest we repeat the mistakes of the past!
One definition of synthetic data, from ChatGPT, reads as follows:
Synthetic data in market research refers to artificially generated data that mimics the characteristics and patterns of real-world data without containing any personally identifiable information (PII) or sensitive information.
It is created through mathematical models and algorithms to stimulate the statistical properties and relationships found in actual datasets. Synthetic data enables researchers to overcome data scarcity issues, maintain privacy compliance, and explore diverse scenarios without relying solely on limited or sensitive real-world data sources.
This allows for more robust analysis, experimentation, and model training while safeguarding privacy and confidentiality.
Put very simply, synthetic data is modelled and weighted data, designed to fill a “gap” in our data references. The source data can be static quantitative data, standard algorithmic output or LLM data, but it remains essentially modelled data.
In the 1980’s, ACNielsen’s Retail Audit in Ireland could not secure the cooperation of one of the country’s biggest retailers, Dunnes Stores. As a result, they took competitor stores of a similar size, turnover, and footfall, added in whatever “hard” sales data certain brands were able to provide, and thereby modelled Dunnes’ sales to provide “synthetic” national and regional estimates. This approach worked very well for high penetration, high purchase frequency brands, but was extremely volatile for low penetration, low purchase frequency brands and for estimating Dunnes own label range. This volatility did occasionally lead to misleading directional guidance, and some very interesting conversations with clients!
Synthetic data is now often presented as a means of (more easily) filling “difficult” sample quotas….researchers have, since MRX began, struggled to cost-effectively and quickly interview “hard to reach” audiences, which synthetic data claims to solve. I personally remember conducting political polling interviews and trying to find 18–21-year-old males on a Friday night, when a football match was playing! Finding a needle in a haystack would have been easier. It was eventually done but it took time and from a commercial perspective, a LOT of money.
The temptation therefore, to see these new applications as miracle solutions or the “holy grail” allowing sample sizes to be achieved and results to be delivered to clients, better, faster, and cheaper (or to exploit the cost savings for commercial gain and improved profits) is clearly huge. BUT…caveat emptor. Life teaches us that when things look to be too good to be true, they often come with risks.
In certain sectors and circumstances, with tightly controlled parameters and a proprietary environment, synthetic data is a welcome development (e.g. “synthetic” cancer patients can be modelled/created on existing data, thus allowing research to be conducted on compliance and adherence to medication, side-effects, disease symptoms etc, using completely anonymised synthetic personas without any personal data or privacy issues).
However, in broader, more complex circumstances (as demonstrated by a study on Land Rover perceptions, presented by Annelies Verhaeghe at ESOMAR’s AI conference in August 2023), the responses provided by the synthetic data were not aligned with real respondent data, and the “model” requires constant updating with “real data” to remain viable and relevant.
This then leads us back to Rigour, triangulation and validation. It is (already) clear that using synthetic respondents in market research can introduce a variety of risks, a topic which has been addressed by a number of authors, one of which is Jason Dunstone’s article from Australia ( https://www.researchsociety.com.au/news-item/16368/embracing-synthetic-datas-potential-while-valuing-real-people).
From our perspective, we consider there to be five key concerns:
Bias and Lack of Representativeness:
Inherent Biases: Synthetic respondents are generated based on models/algorithms that may inadvertently reflect biases present in the training data. This can result in skewed insights that do not accurately represent the target population.
Limited Diversity: Synthetic data may fail to capture the full diversity of real-world respondents, particularly if the algorithms are not sophisticated enough to simulate a wide range of demographics and behaviours.
Quality and Reliability Issues:
Data Quality: The quality of synthetic responses depends heavily on the algorithms and data used to generate them. Poorly designed models can produce low-quality data that does not provide reliable insights.
Validation Challenges: It can be difficult to validate the accuracy and reliability of synthetic data against real-world data, making it hard to ensure the insights derived are trustworthy.
Ethical and Transparency Concerns:
Transparency Issues: The use of synthetic respondents can raise ethical concerns if companies do not transparently communicate their use to stakeholders. This can lead to trust issues with clients and the public.
Ethical Implications: There are ethical considerations around the creation and use of synthetic data, particularly regarding the potential for misuse or manipulation of data to produce desired outcomes.
Impact on Decision Making:
Misguided Decisions: Decisions based on synthetic data that does not accurately reflect real consumer behaviour can lead to misguided strategies and actions, potentially resulting in financial losses and reputational damage.
Over-reliance on Technology: Over-reliance on synthetic data and algorithms (and assuming it will always be “right”), can diminish the importance of human judgement and qualitative insights, which are crucial in understanding complex human behaviours.
Regulatory and Compliance Risks:
Regulatory Challenges: The creation and use of synthetic data may not comply with all regulatory standards, particularly in highly regulated industries. Ensuring compliance with data protection and privacy laws can be complex when using synthetic respondents.
Legal Implications: If synthetic data is found to be non-compliant with legal standards, it can lead to legal challenges and penalties, further complicating the use of such data in market research.
There are three other issues that we also need to be fully aware of:
1) While developments in China remain very opaque, most of the development work in synthetics is in the western, anglophone world, thereby creating a challenge for the inclusion of other countries, languages (LLM’s) and cultures.
2) Even with all the technological developments available today, ensuring fully representative views of minorities and diverse socio-economic groups remains more challenging, and thus the creation of synthetic minority data needs to be very closely reviewed and checked.
3) And finally – and perhaps of most concern - what happens when this (potentially unrepresentative, biased or misguided) synthetic data ends up in Data Lakes (like microplastics into the oceans). In these data warehouses or lakes, once data is combined with multiple other sources and drawn from these lakes in the future for other projects it is entirely plausible that 100% of the sample drawn is compiled of synthetic data with no “real” respondents having been included in the sample. Whilst this may or may not be valid for the project underway, it becomes more important than ever before that the provenance of the data is both known and understood by those interpreting (and using!) the results.
There is a fear that without proper, consistent, identification of synthetic respondents we risk polluting our data lakes in a similar way to how synthetic fibres have polluted our oceans. We would be far better “tagging” these respondents in datasets now, so we can identify them (removing if necessary) in the future rather than, like with microplastics, retrospectively trying to filter then out with limited success.
The Great Pacific Garbage Patch, located between Hawaii and California, is now known and visible to all… will various areas of Data Lakes (datasets) be “garbage” in the future but invisible – with unsuspecting users not realising that the data being extracted is polluted or contaminated with synthetic data/respondents who are (at best) irrelevant, or at worst should be actively excluded from the analysis being conducted?
Finally, this is not to say that there are NO companies trying to do the “right thing” with synthetic data; on the contrary –a great example would be a company like LivePanel, which is definitely trying to buck the trend. However, their efforts do not detract from the far greater pitfalls that will befall us if we do not globally align on mitigating the risks identified above. Equally, you may wish to download Ipsos’ POV article on Synthetic data, published by their Knowledge Centre, which is another balanced view of the role of Synthetic data (https://resources.ipsos.com/Get_Synthetic_Data_POV.html)
Our plea therefore is this .. yes, absolutely, we must embrace new technologies, but we must also be mindful of the risks and be more diligent in trying to predict unintended consequences. Let us ensure NOW that we recognise and mitigate against these challenges through standards (ISO20252), or ethical guidelines or codes of conduct (MRS Code of Conduct, ESOMAR Code), or a standardised global testing scheme which obliges suppliers to share sufficient information with buyers for them to make informed decisions about the data (Ray Poynter webinar, June 2024). Let us put in place the measures and processes to ensure that we can identify synthetic data now, and in the future. Ideally with a joined up, consistent global approach.
A Counterpoint: Stay Calm and test the data!!
People need to calm down about synthetic data. Calls to reject, restrict, or regulate seem to be growing in some quarters of the research industry.
The main argument is that this stuff is at worst fabricated, or at best derived from an opaque soup of training data comprising all of the Most Horrid Things on the internet. Whereas data derived from primary surveys and interviews represents Higher Truth: real, organic, human authenticity.
This binary construct is nonsense.
And at the risk of sounding like Peter Thiel, there’s a real danger of researchers neutering a powerful innovation before we've understood its true character.
We should think of this as 'synthesized' data - as in generated and blended from multiple sources - rather than 'synthetic' in the ersatz, imitative, cheap-and-nasty sense.
Consider these examples.
Glimpse has a feature that allows researchers to ask questions of an individual participant or a segment in a survey dataset. The ‘synthetic’ answers are generated deterministically if they can reasonably be inferred from data captured in the survey, or - if they can’t - probabilistically, with the help of a Large Language Model.
Vurvey gathers video-based responses from hundreds of research participants. The answers are transcribed and used to train ‘agents’ that represent various personas. Researchers can interact with these persona agents to ask questions, test hypotheses and generate ideas.
DeepSights from Market Logic Software uses Retrieval Augmented Generation (RAG) and other methods to synthesize insights from hundreds or thousands of research and data sources - delivering answers to insights-related questions in natural language to stakeholders around an organization.
Are all these creative ways of ‘synthesizing’ insights ‘synthetic’ data? The funny thing about this topic is that the more you learn about it, the less easy it is to define.
I’m going to say we shouldn’t reject these creative use cases for research and insights just because we fear the word ‘synthetic’ or we can’t pin down a tidy definition for it.
We’ve been here before: online surveys, social listening, Big Data - these were all regarded with the same fear and attempts to de-legitimize in the name of defending 'proper' research.
Firstly, synthetic data is not a monolithic thing. It's a broad brush label for a category of data that is generated using a wide range of techniques (LLMs, GANs, RNNs, SMOTE) and a much wider range of data sources (primary research, articles, books, podcasts, blog posts, proprietary documents).
Secondly, the difference between newer forms of synthetic data and established approaches to working with insufficient samples in primary data (imputation, weighting, diffusion modelling, etc.) are not that great.
Thirdly, can we please assess these new techniques on meaningful, objective criteria - rather than a priori assumptions? Do they work? Where do they fall short? What are the benefits and risks? Do they reflect ‘truths’ determined by established research methods? Do they perpetuate or mitigate underlying social and dataset biases? Yogesh Chavda, Ray Poynter, Joel Anderson and others write about these issues thoughtfully, open-mindedly, and - where possible - using evidence.
We have a long way to go, and some synthetic data will inevitably turn out to be snake oil. Some very bad decisions will be made by people who relied on synthetic data. Many of the ‘insights’ based on synthetic data will be bland and uninspiring.
But every use of the term ‘synthetic data’ in the last paragraph could easily be substituted for ‘market research’ today.
So, we should embrace these new methods with an objective, scientific and evidence-driven mindset. Learn where and how they work. Be alert to all the attendant risks - manipulation, misinterpretation, model bias, and others. Ensure responsible disclosure of data sources and methods in all reporting.
But let’s not strangle this thing at birth just because we don't fully understand it yet.
Both of you are missing the point
In 2013 I was attending an MR industry conference in Chicago where I was roped into a workshop about the new technology products beginning to make themselves felt in the market. As debate went back and forth as to the dangers inherent in this new-fangled set of approaches, a young man with a big bushy orange beard stood up and uttered the following immortal words:
“The trouble with researchers is that you know F*&# all about technology. And the trouble with us technologists is that we know F*&# all about research. It’s time we learned about each other.”
Dave Carruthers was that young man – the founder and CEO of VoxPopMe – and he was true to his word. He learned about the industry, educated those in his circle about technology, and paid it both back and forward in terms of his involvement in industry governance and growth.
Dave, however, was part of a ResTech minority. He was wonderful in introducing other technologists to influencers and leaders in the industry, but there were plenty of others who were playing in the industry but were not of it. And it was these to whom we should have been paying attention.
For many entrepreneurs riding a wave of investment, the key to success is to disrupt the industry they are entering. After all, did not Clayton Christensen say that disruptors often came to the party with a product that was initially worse and cheaper than those that the established leaders offered, and then overtook them while they were oblivious?
So it happened in 2010. Except that the leaders and influencers who were oblivious were the national and international associations tasked with governing and defending the industry and its codes of conduct and ethics. It took a full ten years before the key members of the ResTech revolution began to be accepted as legitimate members of the association hierarchy. At which point, the race to the bottom was well under way.
Today, we risk repeating the same story all over again. Many outstanding research companies will be experimenting with Generative AI and ‘synthetic’ data – and will be revealing the results of their experiments in gatherings around the globe, debating the degrees of rigor, transparency and objectivity achieved.
But these are not the values shared by many of the technological entrepreneurs funded by the thundering herd of venture capitalists.
And before you accuse me of “otherism” where such investors are concerned, let’s not forget that they invested over $60 billion in ResTech in twelve years, some of which created real value and innovation and some which wreaked destruction in the form of shit masquerading as research.
Now we face having another wave of tech entrepreneurs and investors who know F*&# all about research. Who will welcome them in? Teach them about the need for ‘rigor, objectivity and transparency’? And introduce them to the world that will depend on them in the future?
If we are successful in welcoming, involving and educating this new generation of insights professionals, all the benefits of AI, including synthetic data, will add real value in terms of having an impact on business performance. But if we leave them out in the wilderness for the next ten years, lack of representativity and quality will lead to bad decisions being made and the ethics of the industry being challenged; and, from there, unwanted (but probably warranted) attention from regulatory authorities.
Or, another race to the bottom.
So, to all leaders, influencers and associations in our beloved industry, I would say this: stretch out your arms and bring these new players inside the tent. Now.
[1] Commonwealth Scientific and Industrial Research Organisation (CSIRO) in Australia
The views expressed by the authors in this publication are not necessarily those of ESOMAR.
Crispin Beale
Chairman at QuMind, CEO at Insight250, Senior Strategic Advisor at mTab, Group President at BehaviorallyCrispin Beale is a marketing, data and customer experience expert. Crispin spent over a decade on the Executive Management Board of Chime Communications as Group CEO of leading brands such as Opinion Leader, Brand Democracy, Facts International and Watermelon. Prior to this Crispin held senior marketing and insight roles at BT, Royal Mail Group and Dixons. Crispin originally qualified as a chartered accountant and moved into management consultancy with Coopers & Lybrand (PwC). Crispin has been a Board Director (and Chairman) of the MRS for nearly 20 years and UK ESOMAR Representative for c15 years. As well as being CEO of Insight250, Crispin is currently Group President of Behaviorally with responsibility for the client and commercial teams globally, the Senior Strategic Advisor at mTab and the Chairman of QuMind. Crispin is a passionate advocate for blending human intelligence and technology to deliver innovation and leadership across organisations.
Simon Chadwick
Managing Partner at Cambiar Consulting, Editor in Chief of Research World at ESOMARSimon founded Cambiar in 2004 to provide strategic assistance to research and insight companies as they face rapid and fundamental change. With 40 years of guiding and managing international organizations of various sizes and stages, Simon’s advice and counsel has helped many companies increase their value – to stakeholders, investors and clients.
He is an acknowledged industry leader, author and conference speaker. In addition to his role at Cambiar, Simon is also a Fellow of the Market Research Society, past Chairman of the Insights Association and Editor-in-Chief of Research World, ESOMAR’s global magazine.
He holds an MA in Philosophy, Politics and Economics from Oxford University, UK, and has done post-graduate studies at both Columbia and Harvard business schools in Change Management and Strategic Management.
Finn Raben
Founder at Amplifi ConsultingWith over 30 years of experience in the insights industry, I have worked across most of the biggest research providers; externally, I have developed successful solutions and strategies for multinational corporates (clients of research), whilst internally, I have been involved in re-organising and restructuring the organisations through the design, implementation and management of change programmes.
For the last 14 years, I have straddled both sides of the industry by heading the global business community of insights and analytics, ESOMAR, as its Director General. In this position at the helm of the worldwide insights sector, I have led our profession through the translation, review, adoption and interpretation of key technological, regulatory and methodological evolutions and obstacles.