Predictive qual
How to turn the art of qual into a science of impact?
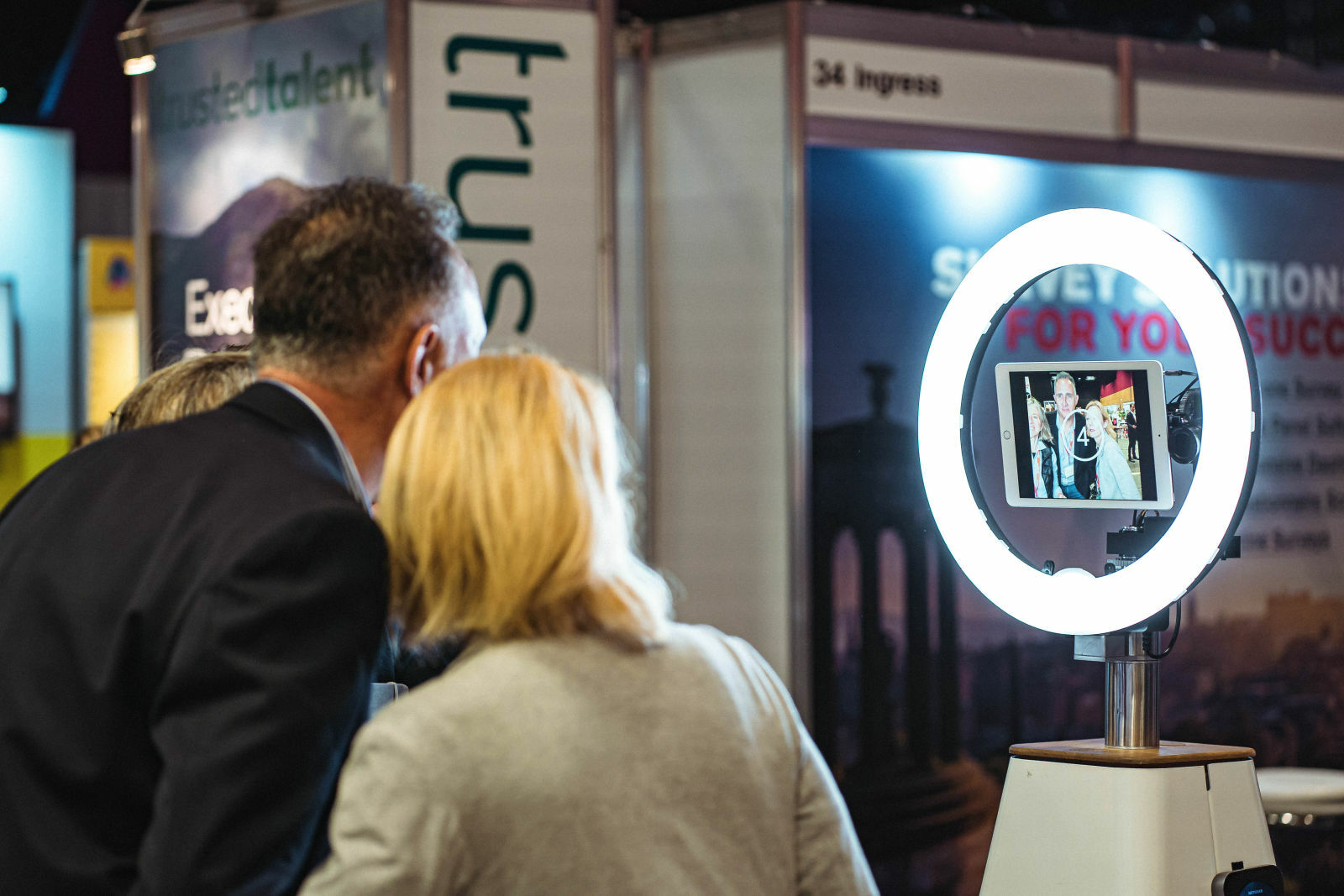
Article series
Qualitative methods
- Safety and (dis)comfort in qualitative research
- Predictive qual
- Insight communities – hidden depths
- Brand ethics in pandemic time
- The hidden key to happiness
- Identity conflict
- Sales and market research
- Practising empathy
- Market research vs. UX research
- What do the futures of quant and qual methodologies hold?
- Social listening has a long way to go
- What I learned in my first 6 months as a market researcher
- Asking questions that will inspire more transformative insights
- Challenges of qualitative research today - Part 1
- Welcome to the Twatterverse!
- Challenges of qualitative research today - Part 2
- Best practices in writing a discussion guide
- Experience improvement
- The post-pandemic dominance of CRM and UX research
It was back in 2017 when David wrote me this email. He was heading insights for SONOS, and the company was bravely acting on their CX Insights. The problem: no improvement after all.
It was a mystery. Nearly 50% of speaker owners were reasoning their loyalty to the great sound. Every other topic was around or way below 10%. So the company was tweaking the sound experience for years. Result: None.
There was no problem in measurement, no serious bias, no categorization mistakes. The data was great. It was telling a clear story.
Still: the story was wrong. Because data is not insight, qualitative feedback is not insight ether.
Our hidden assumption behind this feedback is that customers can answer the question correctly. We assume they are involved and interested enough to dig deep into what is moving their behaviors.
This assumption is like believing humans are rational behaving beings: True only in rare exceptions.
The reality is sobering: customers write something that just comes to their mind. They do not lie, but certainly, they also do not tell the truth either.
As a consequence, what you see across industries that customers are mentioning top-of-mind topics. A speaker owner will say "because of the sound", a washing machine owner will say "washing power", a restaurant customer will reply "great taste of the food", an insurance customer mention the service, and so on.
What's really moving the needle stays hidden.
Is qual useless then?
Not at all. Qual is indispensable. It allows customers to use their own language, words, and expressions. It helps us to discover what we have not thought about.
But here is the question: If this qual feedback -as described above- gives wrong signals. How can this be useful in any sense?
Some would argue that if an argumentation is plausible, it may then be valid. If you can find yourself in this statement, please listen up: this is another GREAT misbelief.
Something plausible has some likelihood to be correct, but everything not plausible can very well be right too.
Qual is a great way of collecting data, perception, customer views, and it is helpful to synthesize new hypotheses. But there is no reliable mechanism to validate it.
The mechanism in use is to check plausibility. In in-depth interviews, you can collect many qualitative data points to check for coherence. But plausibility is a check for coherence that only leverages existing beliefs.
The reason why we do research is that our knowledge is not good enough. We want to learn. Everyone who wants to learn is better off not relying on plausibility.
Qual is broken
Qual is excellent and indispensable. But it is NOT ENOUGH. Even it alone is MISLEADING and can be dangerous.
Why does it seem that I am the first telling you this? Because this is counter-intuitive. A dose of "qual feedback" is like a heroin shot.
You read it; you have thousands of associations with it, you link it with other things you know, and your brain forms a story. Instantly you get "high". The rush of the eureka moment into your brain is hard to resist.
We are all on the needle. My rational me is screaming "noooooooooo", but my heart whispers "yeaaaa" - it automatically beliefs everything that feels plausible.
Predictive qual – is the qual of the AI age.
Imagine a world where we can harness the power of qualitative feedback, but NOW would validate if a mentioned topic is truly causally accountable to explain a specific outcome.
Imagine we could still explore new things that go on in the world of customers and learn about the words they use to express themselves.
But now we would be able to filter out what of those things are just "cheap talk", verbalize the same underlying phenomenon, and the one killer topic that crush outcomes.
What would this mean to CX research, and what would this mean to the entire customer insights and market research discipline?
Wouldn't this mean that suddenly the two worlds of qual and quant do not collide anymore but unit into a coherent approach?
Wouldn't this mean that research becomes even much easier because you can do all -qual and quant and modeling- in one study? Wouldn't this mean that the research process not only becomes BETTER, but also FASTER, and CHEAPER?
How predictive qual works.
Sonos was eager to understand why nothing was working. So we did a deep dive and ran our causal machine learning methodology. It turned out that the sound of SONOS was already good enough, and improving it a waste of resources.
Instead, other good things of the system (e.g. the reliability of the service) were not often mentioned. But when they have been mentioned, it was the killer reason for customer enthusiasm.
With the predictive system behind these insights we even dare to make a prediction. "when you double mentionings of this topic, NPS will increase by 8 points."
Was it a risk? Not if you have a system in place that can explain NPS changes also backward.
Fast forward 6 month of implementation work, suddenly the NPS jumped, precisely by the predicted amount"… a pinch of luck that this was soo precise.
Can you imagine how Leadership got impressed by that?
The magic follows a simple 3 STEP framework that everyone can do themselves
STEP 1: QUANTIFY
Use AI to automate text categorization. Train a supervised AI to achieve optimal accuracy and granularity.
STEP 2: MODEL
Use a key driver analysis (KDA) approach and explain your CX outcome (Satisfaction or Likelihood-to-recommend) to the topics mentioned and the verbatim's sentiment. For better results, use causal machine learning (instead of KDA) for doubling predictive and prescriptive power.
STEP 3: VISUALIZE & PREDICT
Visualized results in an interactive dashboard so everyone can play with it. Use the driver model formula to predict simulated changes in drivers onto outcomes.
The predictive qual trend.
The trend towards "predictive qual" is apparent. Many CX software and insights platforms integrated not only text analytics (step 1) but also a driver analysis (step 2). It's a clear sign that pioneers among insights leaders are already adopting this methodology.
It fills me with a little bit of pride, having started this movement back then in 2017. In truth, David from Sonos was the "stone" who got the line started.
Soon after, big brands like Microsoft followed and helped us to build with CX-AI.com the best Predictive Qual method available – providing 4X impact of actions compared to DIY solutions.
In a nutshell
Qualitative feedback is indispensable. It gets us unfiltered feedback and enables us to discover new things.
But just reading it can be largely misleading. It's even a proven fact that just counting the number of mentioned topics (as a reason for satisfaction or loyalty) is not related to its importance.
To draw adequate conclusions from unstructured feedback, it takes a Predictive Qual approach.
The approach can be filled with a three-step framework: text analytics, driver analytics, and predictive dashboarding.
With state-of-the-art excellence in each step, you can 4X the impact of the action that will be derived from the data.
This means it matters HOW you implement it.
Dr. Frank Buckler
Founder & CEO at Success DriversArticle series
Qualitative methods
- Safety and (dis)comfort in qualitative research
- Predictive qual
- Insight communities – hidden depths
- Brand ethics in pandemic time
- The hidden key to happiness
- Identity conflict
- Sales and market research
- Practising empathy
- Market research vs. UX research
- What do the futures of quant and qual methodologies hold?
- Social listening has a long way to go
- What I learned in my first 6 months as a market researcher
- Asking questions that will inspire more transformative insights
- Challenges of qualitative research today - Part 1
- Welcome to the Twatterverse!
- Challenges of qualitative research today - Part 2
- Best practices in writing a discussion guide
- Experience improvement
- The post-pandemic dominance of CRM and UX research